Deep Learning for Microscopy Image Analysis 2021
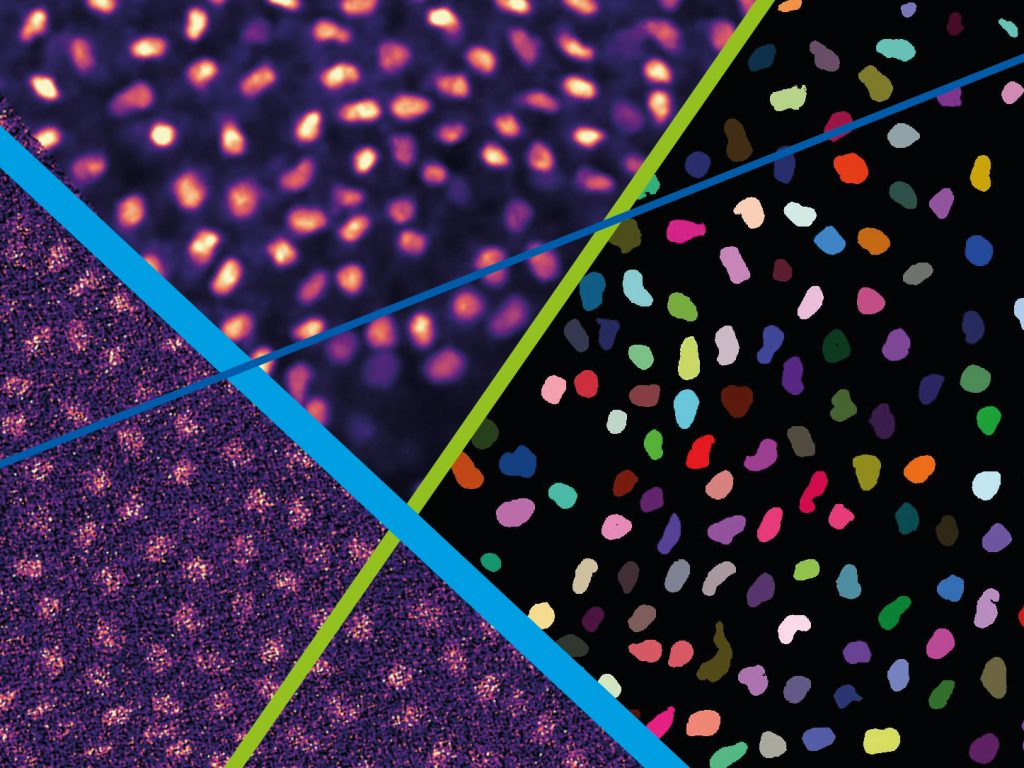
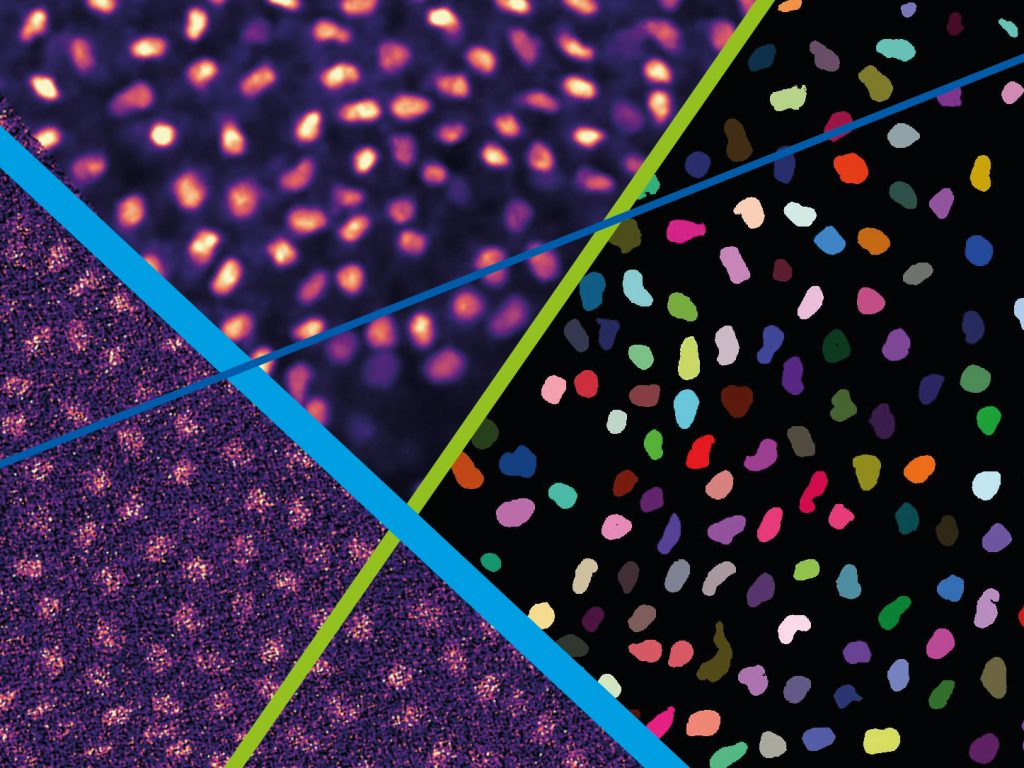
Category: Course / Workshop
Location: Online
Registration Deadline: 31/10/2021
Date: 22/11/2021 - 26/11/2021
Target Audience: Up to 24 students who are expected to have coding/scripting skills and some familiarity with Python programming, with no necessary prior experience with machine learning or deep learning techniques. Students are strongly encouraged to bring their own microscopy datasets to work on during the project phase.
AIM OF THE COURSE
The goal of this course is to familiarize researchers working in life sciences with state-of-the-art deep learning techniques for microscopy image analysis, with a focus on image restoration and image segmentation. Our aim is to introduce tools and frameworks that will facilitate independent application of the learned material after the course.
The following topics will be covered extensively during lectures, exercises, and project work:
- image denoising and restoration (fully supervised, self-supervised and unsupervised machine learning),
- image segmentation (pixel classification, instance segmentation, shallow and deep approaches),
- failure cases and limitations.
The course will be organised in two phases: (1) First three days with lectures and exercises to introduce participants to the basic concepts of deep learning and familiarize them with the methods and tools. (2) Last two days with hands-on projects, where students will work together and with trainers to apply the newly acquired skills to their own datasets.
Students will leave the course with an appreciation for the power and limitations of deep learning, as well as with helpful insights into the underlying theory of machine learning techniques and the most prevalent tools for design and training of neural networks.
BRIEF DESCRIPTION
Students (max 24) will learn about recently developed deep learning software packages used to circumvent limitations in microscopy and obtain useful quantitative microscopy images.
COURSE REQUIREMENTS
Students will work on virtual machines and need access to a computer with high-speed internet connection. The course requires a Zoom installation.
Contact: training@fht.org
Lecturers
-
Florian Jug
Research Group Leader, Human Technopole, IT -
Anna Kreshuk
Group Leader, EMBL, DE -
Alexander Krull
Lecturer in Data Science and AI, University of Birmingham, UK -
Loïc Royer
Group Leader, CZ Biohub, US -
Virginie Uhlmann
Group Leader, EMBL-EBI, UK -
Martin Weigert
Group Leader, EPFL, CH
HT Trainers
-
Florian Jug
Research Group Leader, HT -
-
Joran Deschamps
Image Analysis Researcher and Research Software Engineer Coordinator, HT
Scientific Organiser
-
Florian Jug
Research Group Leader, HT