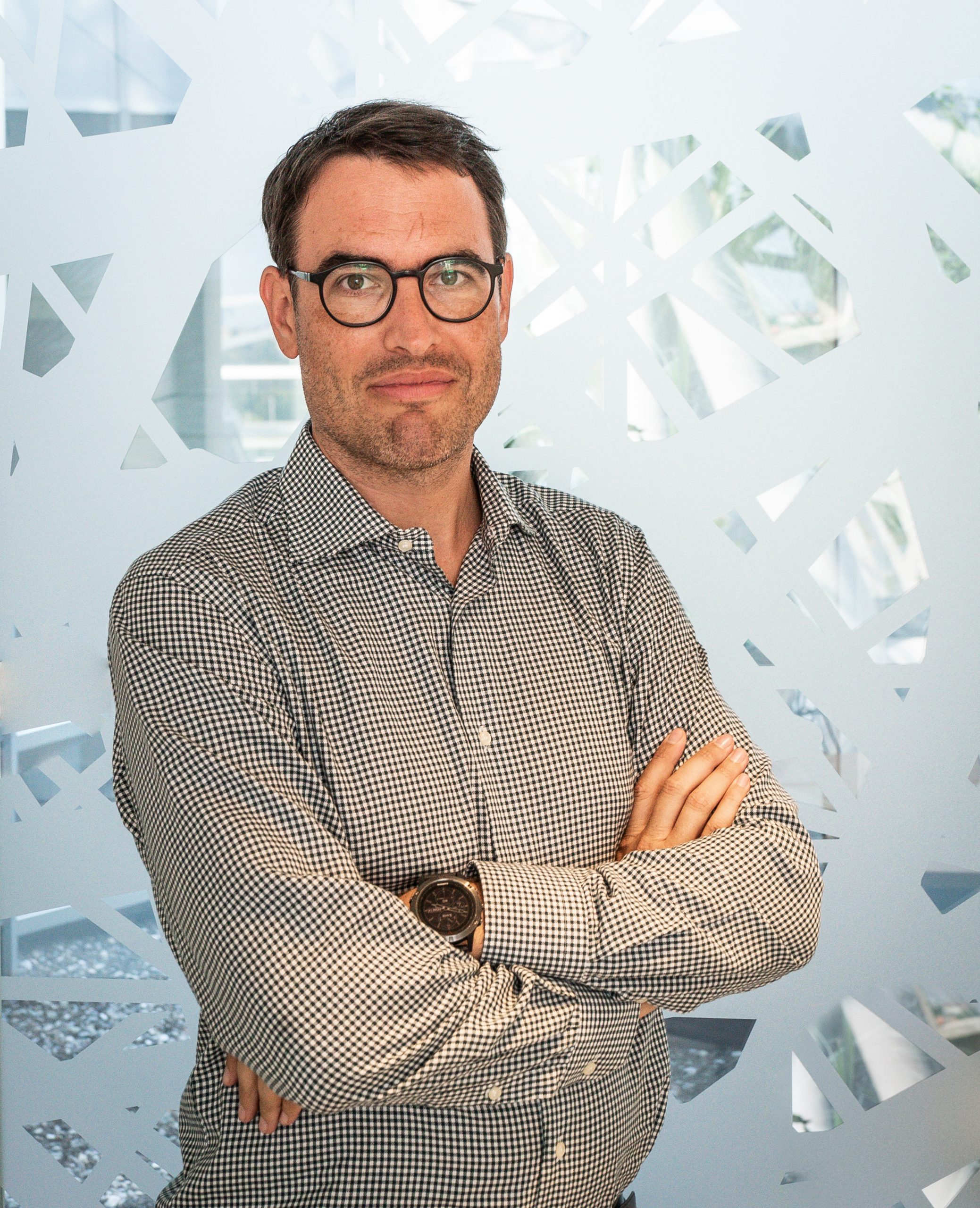
Florian Jug
- Head of Image Analysis Facility, Computational biology
- Research Group Leader, Jug Group
Dr. Florian Jug holds a PhD in Computational Neuroscience from the Institute of Theoretical Computer Science at ETH Zurich. His research aims at pushing the boundary of what AI and machine learning can do to better analyze and quantify biological data. At HT, Dr. Jug covers the full breadth of bio-image computing, from research on novel methods for computer vision and machine learning, all the way to offering bio-image analysis as a service.
Florian Jug is a strong proponent of open access science, open AI and ML methods, and open source software. His team is a core contributor to Fiji (~100,000 active users) and collaboratively develops open methods such as CARE, Noise2Void, PN2V, DivNoising, etc. He organizes scientific conferences (e.g the I2K conference), workshops (e.g. the BIC workshops at top-tier computer vision conferences) and various practical courses on machine learning for bio-image analysis (e.g. DL@MBL in Woods Hole) or microscopy (e.g. Quantitative Imaging at Cold Spring Harbor).
Contacts
Follow on
Publications
-
08/2020 - BioRxiv
ZeroCostDL4Mic: an open platform to use Deep-Learning in Microscopy
The resources and expertise needed to use Deep Learning (DL) in bioimaging remain significant barriers for most laboratories. We present https://github.com/HenriquesLab/ZeroCostDL4Mic/wiki, a platform simplifying access to DL by exploiting the free, cloud-based computational resources of Google Colab. https://github.com/HenriquesLab/ZeroCostDL4Mic/wiki allows researchers to train, evaluate, and apply key DL networks to perform tasks including segmentation, detection, denoising, restoration, resolution enhancement […]
-
06/2020 - arXiv
DivNoising: Diversity Denoising with Fully Convolutional Variational Autoencoders
Deep Learning based methods have emerged as the indisputable leaders for virtually all image restoration tasks. Especially in the domain of microscopy images, various content-aware image restoration (CARE) approaches are now used to improve the interpretability of acquired data. But there are limitations to what can be restored in corrupted images, and any given method […]
-
06/2020 - arXiv
DenoiSeg: Joint Denoising and Segmentation
Microscopy image analysis often requires the segmentation of objects, but training data for this task is typically scarce and hard to obtain. Here we propose DenoiSeg, a new method that can be trained end-to-end on only a few annotated ground truth segmentations. We achieve this by extending Noise2Void, a self-supervised denoising scheme that can be […]
-
07/2019 - Methods in Cell Biology
Content-aware image restoration for electron microscopy
Multiple approaches to use deep neural networks for image restoration have recently been proposed. Training such networks requires well registered pairs of high and low-quality images. While this is easily achievable for many imaging modalities, e.g., fluorescence light microscopy, for others it is not. Here we summarize on a number of recent developments in the […]
-
10/2018 - ArXiv
Cryo-CARE: Content-Aware Image Restoration for Cryo-Transmission Electron Microscopy Data
Multiple approaches to use deep learning for image restoration have recently been proposed. Training such approaches requires well registered pairs of high and low quality images. While this is easily achievable for many imaging modalities, e.g. fluorescence light microscopy, for others it is not. Cryo-transmission electron microscopy (cryo-TEM) could profoundly benefit from improved denoising methods, […]