Machine learning reveals hidden features in histology images
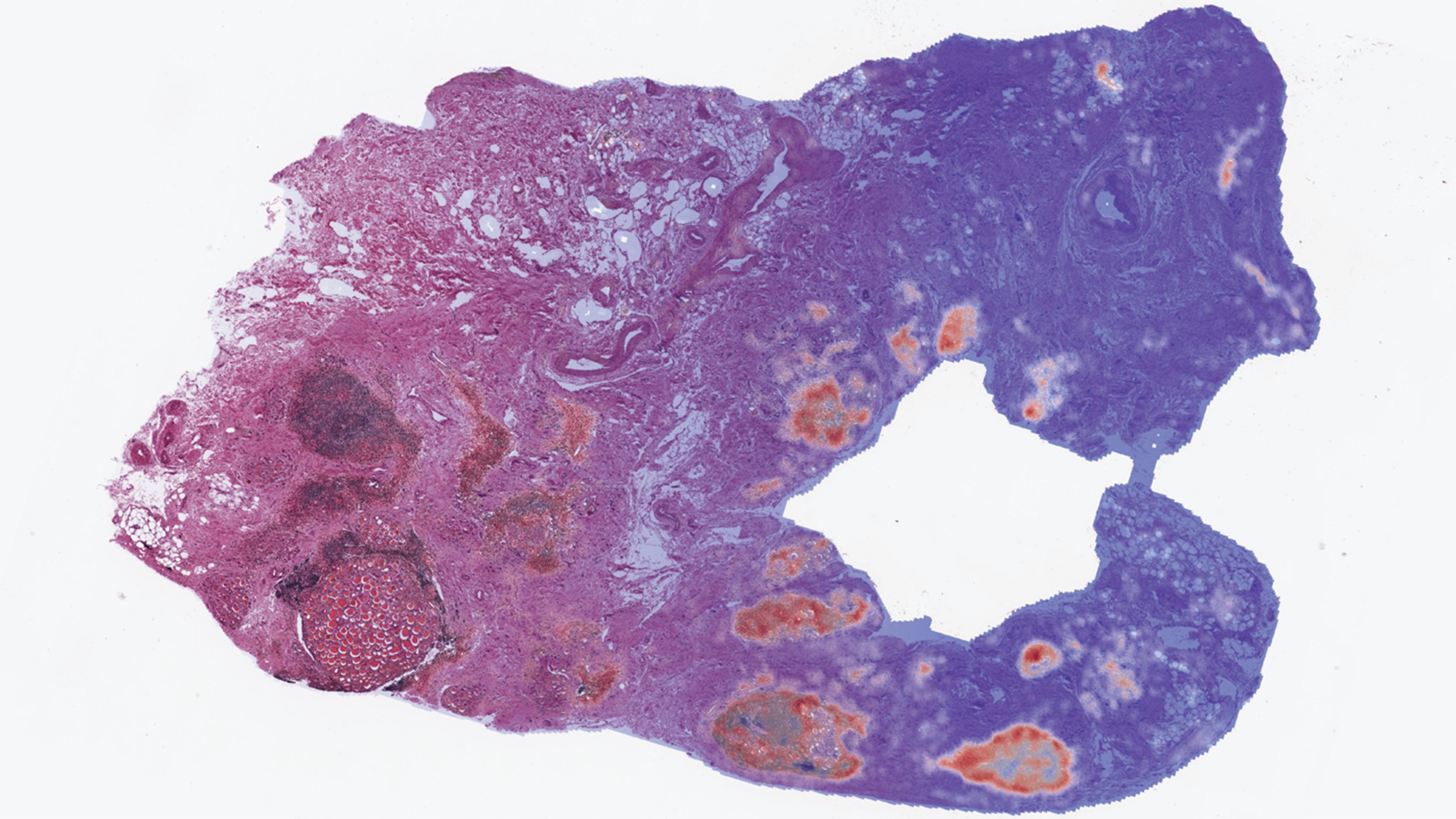
Researchers at Human Technopole developed a self-supervised machine learning model that combines histology, gene expression, and genetic variation to automatically identify and cluster distinct tissue substructures, cells, and pathological features in human tissues.
Histology is a technique that allows microscopic identification of different cellular components and structures in a tissue. Histological examination of tissues is paramount for accurately diagnosing diseases and provides crucial information in clincal diagnoses. Traditionally, pathologists examine stained tissue sections under a microscope. However, the advent of digitalisation and computational methods have made it possible to scan histology images at high resolution and to automatically analyse them using machine learning-based approaches. Recently, efforts have been made to match histology and molecular data, such as large RNA sequencing and Whole Genome Sequencing datasets, from thousands of samples. Combining this information would give important insights into how tissue structure and function vary in a population and how genetic variation and gene expression impact healthy and diseased tissues.
Research conducted by Francesco Cisternino, a PhD student in the lab of Dr Craig A. Glastonbury (The Glastonbury Group) at the Human Technopole Genomics Research Centre has led to the development of a new machine learning model based on Vision Transformers (ViT) that learns to cluster and segment tissue automatically. The researchers combined histology, gene expression, and genetic variation data in more than 13,000 samples representative of 23 healthy human tissues from 838 donors.
The study has now been published in Nature Communications.
By analysing gigapixel Whole Slide Images, the Group found significant intra-tissue variability across donors and identified unannotated pathologies such as calcification events, incorrect tissue assignment and tissue contamination. In addition, they discovered gene expression signatures of specific tissue substructures and revealed previously unknown genetic associations.
The researchers also developed RNAPath, a machine learning model that enables them to predict and spatialise gene expression levels from H&E histology images alone. RNAPath outperformed other competing methods, such as HE2RNA, a widely used deep learning model to predict RNA-Seq expression from whole slide images.
In summary, this study reveals that self-supervised machine learning methods and histological archives can be used to learn new insights into disease pathology and tissue organisation and allows researchers to explore the interplay between morphological tissue variability and gene expression.
The research lead Craig Glastonbury commented, “As histological archives and pathology workflows become digital, we believe there is substantial opportunity for using self-supervised learning to uncover novel, fundamental biology about tissue structure, function and its variability in a population in both healthy and diseased subjects”.
Cisternino, F., Ometto, S., Chatterjee, S. et al. Self-supervised learning for characterising histomorphological diversity and spatial RNA expression prediction across 23 human tissue types. Nat Commun 15, 5906 (2024). https://doi.org/10.1038/s41467-024-50317-w
Image: RNAPath predicting the spatial location of CD19 expression across a H&E thyroid tissue section.