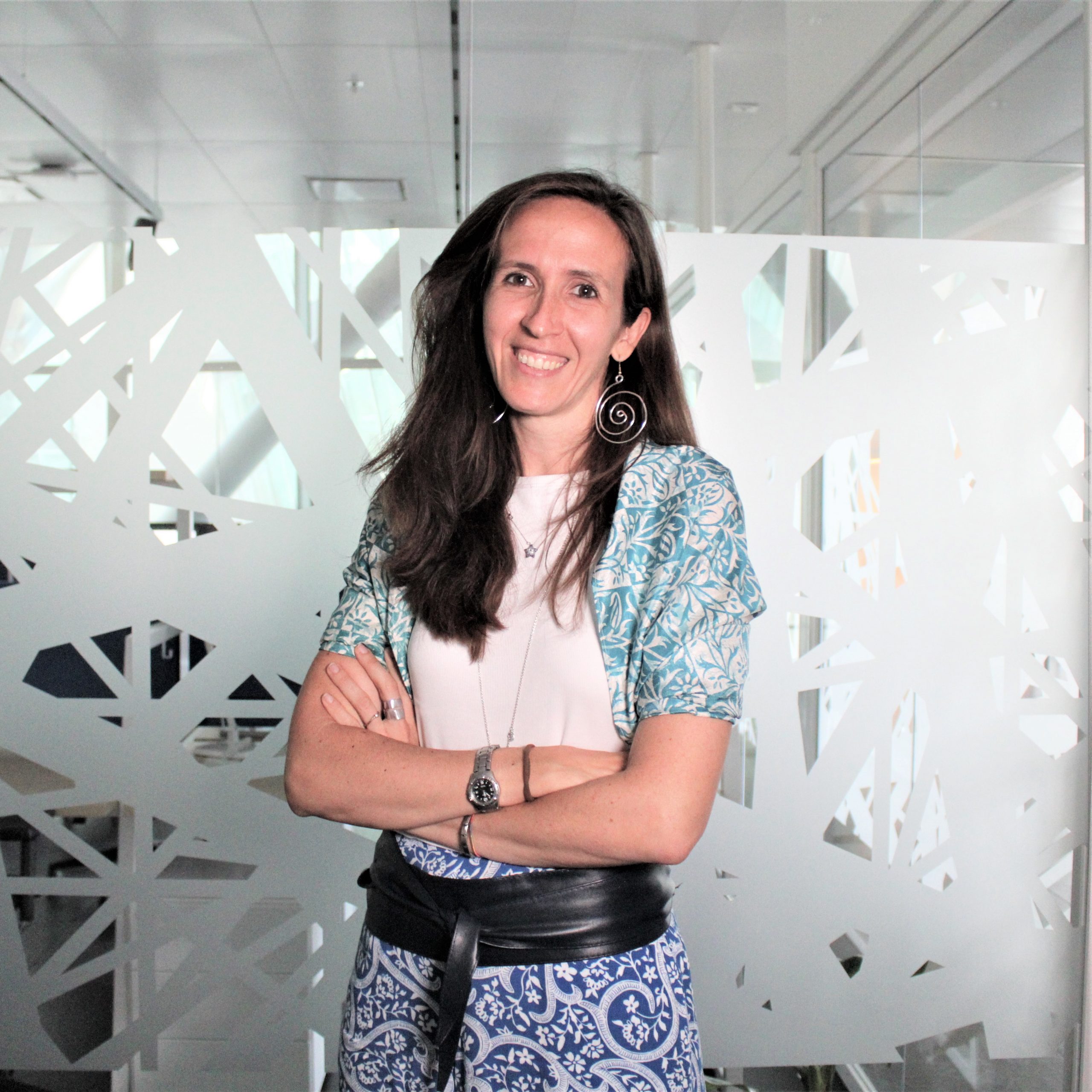
Francesca Ieva
- Associate Head of Research centre, Health Data Science
- Associate Professor of Statistics at MOX - Modeling and Scientific Computing laboratory, Department of Mathematics, Politecnico di Milano, Health Data Science
- Group Leader, Di Angelantonio & Ieva Group
Francesca Ieva is Associate Head of the Health Data Science Centre at Human Technopole and Professor of Statistics at Politecnico di Milano.
She got a PhD in Mathematical Models and Methods for Engineering in 2012.
Her research focuses on statistical learning in biomedical context, and the development of advanced models for complex clinical data integration, to inform predictions in clinical decision making, and to support precision medicine and precision policies
Follow on
Top 10 Publications
-
06/2020 - BMC Health Services Research
Evaluating the effect of healthcare providers on the clinical path of heart failure patients through a semi-Markov, multi-state model
Background Investigating similarities and differences among healthcare providers, on the basis of patient healthcare experience, is of interest for policy making. Availability of high quality, routine health databases allows a more detailed analysis of performance across multiple outcomes, but requires appropriate statistical methodology. Methods Motivated by analysis of a clinical administrative database of 42,871 Heart […]
-
11/2019 - Scientific Reports
Comparing methods for comparing networks
With the impressive growth of available data and the flexibility of network modelling, the problem of devising effective quantitative methods for the comparison of networks arises. Plenty of such methods have been designed to accomplish this task: most of them deal with undirected and unweighted networks only, but a few are capable of handling directed […]
-
12/2018 - Biostatistics
Nonparametric frailty Cox models for hierarchical time-to-event data
We propose a novel model for hierarchical time-to-event data, for example, healthcare data in which patients are grouped by their healthcare provider. The most common model for this kind of data is the Cox proportional hazard model, with frailties that are common to patients in the same group and given a parametric distribution. We relax […]
-
07/2016 - BMC Health Services Research
Methodological issues on the use of administrative data in healthcare research: the case of heart failure hospitalizations in Lombardy region, 2000 to 2012
Background Administrative data are increasingly used in healthcare research. However, in order to avoid biases, their use requires careful study planning. This paper describes the methodological principles and criteria used in a study on epidemiology, outcomes and process of care of patients hospitalized for heart failure (HF) in the largest Italian Region, from 2000 to […]
-
03/2015 - Statistical Methods in Medical Research
Multi-state modelling of repeated hospitalisation and death in patients with heart failure: The use of large administrative databases in clinical epidemiology
In chronic diseases like heart failure (HF), the disease course and associated clinical event histories for the patient population vary widely. To improve understanding of the prognosis of patients and enable health care providers to assess and manage resources, we wish to jointly model disease progression, mortality and their relation with patient characteristics. We show […]