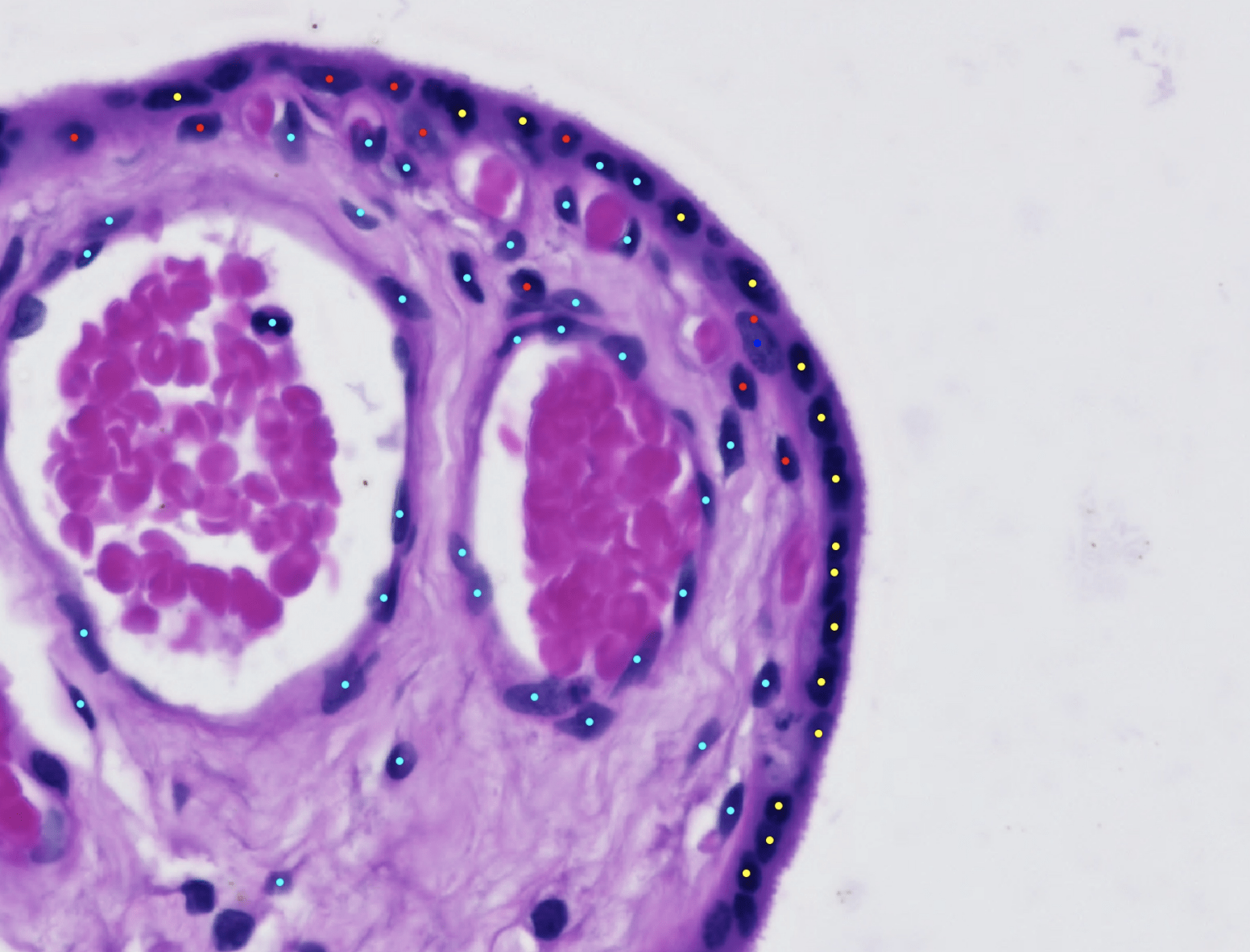
Glastonbury Group
The Glastonbury Group applies and develops machine learning methods to understand the genetic basis of a range of complex diseases. With the advent of population scale biobanking, rich data modalities are being collected on hundreds of thousands of individuals. One such data type is imaging data, a high dimensional and information rich modality for human phenotyping.
The Glastonbury Group is interested in applying machine learning techniques to any aspect of human genetic discovery (Variant → Gene → Phenotype). One of the key focuses the Glastonbury Group has is to extract cellular and disease mechanism phenotypes from histopathology images. Whole Slide Imaging (WSI) of tissue sections are routinely used to diagnose a range of diseases, such as Inflammatory Bowel Disease (IBD), various oncologies as well as neurological diseases post-mortem. By using both supervised and unsupervised machine learning techniques, it is possible to extract highly precise cellular and tissue phenotypes from such images and use them to characterise the cause and consequence of an underlying disease. By coupling such image derived phenotypes with genetic information (e.g. genotyping, exome, whole genome-sequencing), we can start to unpick mechanistically how a genetic variant contributes to increasing disease risk and how such risk is shared across diseases and phenotypes.
The Glastonbury Group is recruiting for PhD’s, Postdoctoral Positions, and a staff scientist. Please get in touch [craig.glastonbury{at}.fht.org] if you’re interested in any of the following topics:
- Machine learning method development (unsupervised, self-supervised, supervised) & application to large scale biomedical imaging datasets
- Unsupervised Phenotype discovery using clinical data
- Development of scalable methods and pipelines for rapid GWAS and gene prioritisation
Group members
-
Craig Glastonbury
Research Group Leader -
Emanuele Bianchi
Undergraduate Intern -
Soumick Chatterjee
Postdoc -
Francesco Cisternino
PhD Student -
Rupali Khatun
Scientific Visitor -
Sara Ometto
Bioinformatician -
Emanuel Soda
PhD Student