Introducing Craig Glastonbury
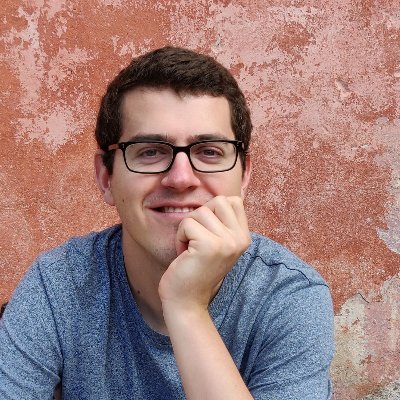
Craig Glastonbury is a geneticist with a background in Machine Learning applied to biological problems. His group’s core scientific interest is to understand the genetic and mechanistic basis of complex diseases. To do this, Craig combines the latest developments in Machine Learning with large scale genomic and clinical datasets, with a specific focus on histopathology and medical imaging.
Craig Glastonbury is currently a Lead Machine Learning Researcher at BenevolentAI, London UK, where he leads the genetics squad in the Precision Medicine team. Before working in Industry, Craig was a postdoctoral researcher at the Big Data Institute (BDI) at the University of Oxford, and previously completed his PhD at King’s College London. Craig will be joining Human Technopole in May 2022, where he will establish a research group in the Genomics Research Centre – Medical & Population Genomics Programme. The Glastonbury Group will explore the use and development of scalable Machine Learning methods applied to large-scale imaging and genetic cohorts to understand the mechanistic basis of GWAS loci in a range of complex diseases.
Did you always know you wanted to be a scientist?
I’m not sure I always wanted to be a scientist, but I did know from my early teens that I was fascinated by science, from physics to evolutionary biology. I remember finding it difficult to choose between computer science or biology at university, so to now find myself at the intersection of both, is great! Science as a career is a real privilege, it’s such an interesting and fulfilling job and I’m lucky to be able to pursue my own scientific research programme at Human Technopole.
What will you be working on at the Genomics Research Centre?
My group most broadly will be looking at using Machine Learning to understand the mechanistic and genetic basis of complex disease. More specifically, a key focus will be working on how to extract phenotypes or diagnostic characteristics from large-scale medical imaging datasets using both supervised and unsupervised ML techniques. By coupling image derived phenotypes with genetics, we will be able to start understanding the cellular and mechanistic basis to a range of complex diseases.
Tell us a bit more about your research. What is the most fascinating aspect of it?
I think it’s the interface between Machine Learning and biology. Both fields are growing at such a rapid rate, it’s extremely exciting, but also sometimes difficult to keep fully abreast of both fields. However, taking the latest developments from the Machine Learning community, and applying them to important biological questions, that’s what really excites me. For example, self-supervised learning, a form of unsupervised learning, has started to reach similar performance to fully-supervised algorithms. I think this field in particular has great promise in computational biology, where labels are expensive to obtain but there are vast amounts of data.
How did you learn about Human Technopole and what inspired you to apply?
I was familiar with Nicole Soranzo and her group at the Sanger Institute, so when I saw the position being advertised, I was really impressed about the calibre of scientists HT had recruited. HT has built-up facilities that really complement my work, from the Genomics centre to our dedicated Imaging facilities. So, this combined with me wanting to move back to academia to start my own group, and work in another country, ticked all the boxes.
Research can have many ups and downs, big successes but also disappointing moments. How do you find motivation when it is lacking?
Sometimes failed projects or rejected papers can be very demotivating. I find looking at the bigger picture helps me. Why am I working on this? Why does it matter? If you have answers to those questions, you can overlook short-term and often unimportant failures. Also, science isn’t always about the individual, so through mentorship and collaboration, I get to see and help others succeed which is hugely rewarding in itself.