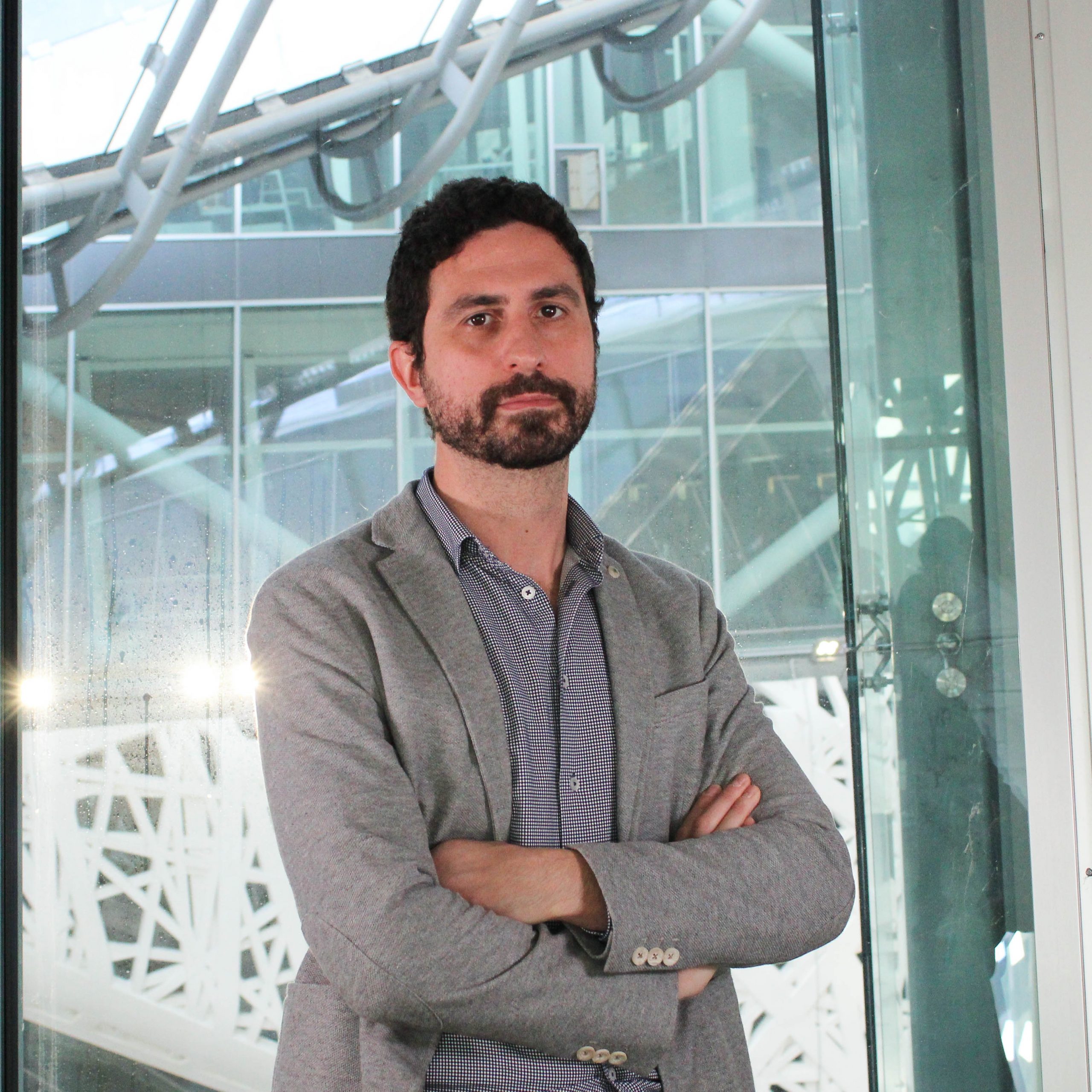
Andrea Sottoriva
- Head of Computational Biology Research Centre, Computational biology
- Research Group Leader, Sottoriva Group
Andrea Sottoriva is the Head of the Computational Biology Research Centre at Human Technopole.
Andrea’s research focusses on the development of new computational approaches to measure cancer evolution in patients, with the aim of predicting the future course of the disease. Andrea’s lab also integrates patient-derived experimental models and multiomics data, with evolutionary methods to design new treatment strategies that aim at preventing and controlling drug resistance.
After graduating in Computer Science at the University of Bologna in 2006, he obtained a master in Computational Sciences from the University of Amsterdam in 2008. During his studies, he worked in neutrino physics at the Department of Physics of the University of Bologna and at the Institute for Nuclear and High Energy Physics (NIKHEF) in the Netherlands as a research assistant.
In 2012 he completed his PhD in Computational Biology from the University of Cambridge, where he worked at the Cancer Research UK research centre.
After postdoctoral work at the University of Southern California, he started his lab at the Institute of Cancer Research in London in 2013, where in 2018 he became the Deputy Director of the Centre for Evolution and Cancer and then the Director in 2020.
He authored several studies published in prestigious scientific journals, including Science, Nature, Nature Genetics and Cancer Discovery. Among his articles are “The co-evolution of the genome and epigenome in colorectal cancer” (Nature, 2022), “Phenotypic plasticity and genetic control in colorectal cancer evolution” (Nature, 2022), “Subclonal reconstruction of tumors by using machine learning and population genetics” (Nature Genetics, 2020), “Detecting repeated cancer evolution from multi-region tumor sequencing data” (Nature Methods, 2018), “Longitudinal liquid biopsy and mathematical modelling of clonal evolution forecast waiting time to treatment failure in a phase II colorectal cancer clinical trial” (Cancer Discovery, 2018), and “Patient-derived organoids model treatment response of metastatic gastrointestinal cancers” (Science, 2018).
In 2016 he was awarded the Cancer Research UK Future Leaders in Cancer Research prize.
Contacts
Follow on
Selected Publications
-
02/2020 - Nature Communications
Measuring single cell divisions in human tissues from multi-region sequencing data
Both normal tissue development and cancer growth are driven by a branching process of cell division and mutation accumulation that leads to intra-tissue genetic heterogeneity. However, quantifying somatic evolution in humans remains challenging. Here, we show that multi-sample genomic data from a single time point of normal and cancer tissues contains information on single-cell divisions. […]
-
07/2019 - PloS CompBio
Spatially constrained tumour growth affects the patterns of clonal selection and neutral drift in cancer genomic data
Quantification of the effect of spatial tumour sampling on the patterns of mutations detected in next-generation sequencing data is largely lacking. Here we use a spatial stochastic cellular automaton model of tumour growth that accounts for somatic mutations, selection, drift and spatial constraints, to simulate multi-region sequencing data derived from spatial sampling of a neoplasm. […]
-
07/2019 - Nature Reviews Genetics
Resolving genetic heterogeneity in cancer
To a large extent, cancer conforms to evolutionary rules defined by the rates at which clones mutate, adapt and grow. Next-generation sequencing has provided a snapshot of the genetic landscape of most cancer types, and cancer genomics approaches are driving new insights into cancer evolutionary patterns in time and space. In contrast to species evolution, […]
-
10/2018 - Cancer Discovery
Longitudinal Liquid Biopsy and Mathematical Modeling of Clonal Evolution Forecast Time to Treatment Failure in the PROSPECT-C Phase II Colorectal Cancer Clinical Trial
Sequential profiling of plasma cell-free DNA (cfDNA) holds immense promise for early detection of patient progression. However, how to exploit the predictive power of cfDNA as a liquid biopsy in the clinic remains unclear. RAS pathway aberrations can be tracked in cfDNA to monitor resistance to anti-EGFR monoclonal antibodies in patients with metastatic colorectal cancer. […]
-
08/2018 - Nature Methods
Detecting repeated cancer evolution from multi-region tumor sequencing data
Recurrent successions of genomic changes, both within and between patients, reflect repeated evolutionary processes that are valuable for the anticipation of cancer progression. Multi-region sequencing allows the temporal order of some genomic changes in a tumor to be inferred, but the robust identification of repeated evolution across patients remains a challenge. We developed a machine-learning […]