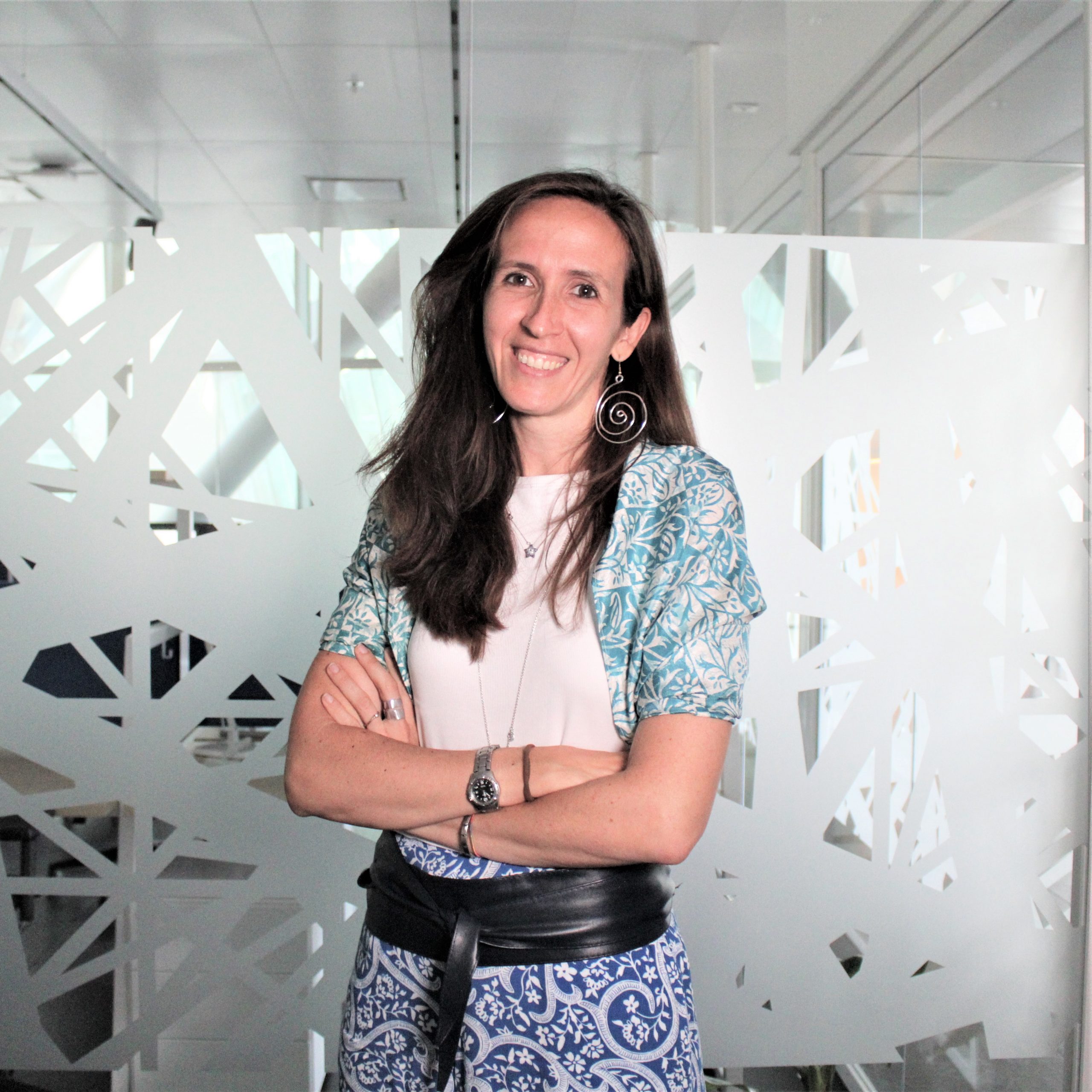
Francesca Ieva
- Associate Head of Research centre, Health Data Science
- Associate Professor of Statistics at MOX - Modeling and Scientific Computing laboratory, Department of Mathematics, Politecnico di Milano, Health Data Science
- Group Leader, Di Angelantonio & Ieva Group
Francesca Ieva is Associate Head of the Health Data Science Centre at Human Technopole and Professor of Statistics at Politecnico di Milano.
She got a PhD in Mathematical Models and Methods for Engineering in 2012.
Her research focuses on statistical learning in biomedical context, and the development of advanced models for complex clinical data integration, to inform predictions in clinical decision making, and to support precision medicine and precision policies
Contacts
Follow on
Top 10 Publications
-
09/2022 - Clinical Epigenetics
A blood DNA methylation biomarker for predicting short-term risk of cardiovascular events
Background Recent evidence highlights the epidemiological value of blood DNA methylation (DNAm) as surrogate biomarker for exposure to risk factors for non-communicable diseases (NCD). DNAm surrogate of exposures predicts diseases and longevity better than self-reported or measured exposures in many cases. Consequently, disease prediction models based on blood DNAm surrogates may outperform current state-of-the-art prediction […]
-
09/2022 - PloS CompBio
Deep Survival EWAS approach estimating risk profile based on pre-diagnostic DNA methylation: an application to Breast Cancer time to diagnosis
Previous studies for cancer biomarker discovery based on pre-diagnostic blood DNA methylation profiles, either ignore the explicit modeling of the time to diagnosis (TTD) as in a survival analysis setting, or provide inconsistent results. This lack of consistency is likely due to the limitations of standard EWAS approaches, that model the effect of DNAm at […]
-
08/2021 - Radiotherapy & Oncology
PH-0656 Prediction of toxicity after prostate cancer RT: the value of a SNP-interaction polygenic risk score
-
08/2020 - Biometrical Journal
Dynamic monitoring of the effects of adherence to medication on survival in heart failure patients: A joint modeling approach exploiting time-varying covariates
Adherence to medication is the process by which patients take their drugs as prescribed, and represents an issue in pharmacoepidemiological studies. Poor adherence is often associated with adverse health conditions and outcomes, especially in case of chronic diseases such as heart failure (HF). This turns out in an increased request for health care services, and […]