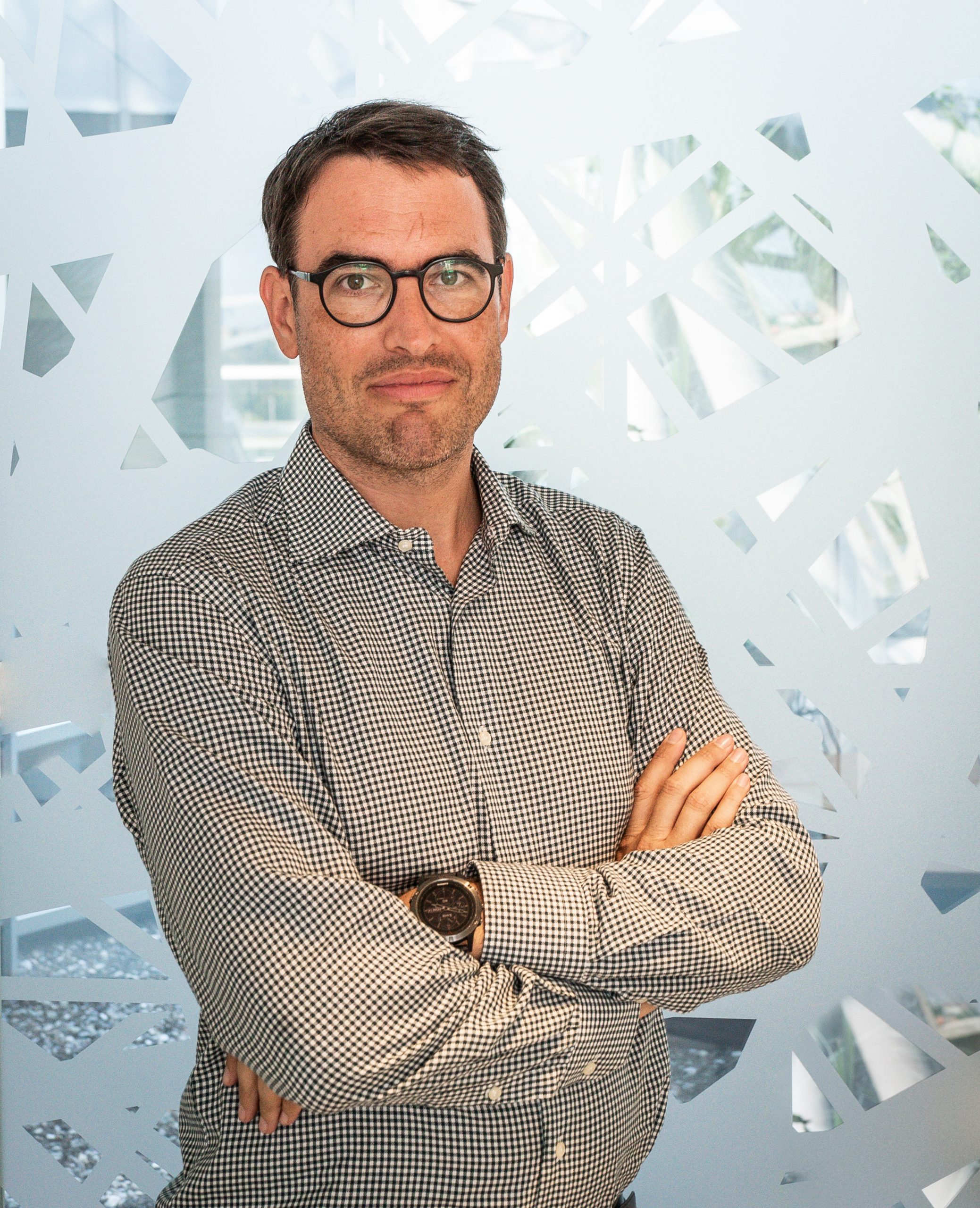
Florian Jug
- Head of Image Analysis Facility, Biologia Computazionale
- Research Group Leader, Jug Group
Il dott. Florian Jug ha conseguito un dottorato in neuroscienze computazionali presso l’Institute of Theoretical Computer Science dell’ETH di Zurigo. La sua ricerca mira a superare i confini di ciò che l’intelligenza artificiale e l’apprendimento automatico possono fare per analizzare e quantificare meglio i dati biologici. In HT, il dottor Jug copre l’intera gamma del calcolo delle bio-immagini, dalla ricerca su nuovi metodi per la visione artificiale e l’apprendimento automatico, fino al servizio di analisi di bio-immagini.
Florian Jug è un forte sostenitore della scienza open access, grazie anche alla condivisione di metodi ML e di intelligenza artificiale e software open source. Il suo team da un forte contributo al software Fiji (circa 100.000 user attivi) e sviluppa in modo collaborativo metodi quali CARE, Noise2Void, PN2V, DivNoising ecc. Organizza conferenze scientifiche (per es. la conferenza I2K), workshop (per es. i workshop BIC a conferenze di alto livello in tema di computer vision) e vari corsi di formazione pratici sull’apprendimento automatico per l’analisi di bio immagini (per es. DL@MBL a Woods Hole) o sulla microscopia (per es. Quantitative Imaging a Cold Spring Harboe).
Email: florian.jug[at]fht.org
Contatti
Segui
Pubblicazioni
-
08/2020 - BioRxiv
ZeroCostDL4Mic: an open platform to use Deep-Learning in Microscopy
The resources and expertise needed to use Deep Learning (DL) in bioimaging remain significant barriers for most laboratories. We present https://github.com/HenriquesLab/ZeroCostDL4Mic/wiki, a platform simplifying access to DL by exploiting the free, cloud-based computational resources of Google Colab. https://github.com/HenriquesLab/ZeroCostDL4Mic/wiki allows researchers to train, evaluate, and apply key DL networks to perform tasks including segmentation, detection, denoising, restoration, resolution enhancement […]
-
06/2020 - arXiv
DivNoising: Diversity Denoising with Fully Convolutional Variational Autoencoders
Deep Learning based methods have emerged as the indisputable leaders for virtually all image restoration tasks. Especially in the domain of microscopy images, various content-aware image restoration (CARE) approaches are now used to improve the interpretability of acquired data. But there are limitations to what can be restored in corrupted images, and any given method […]
-
06/2020 - arXiv
DenoiSeg: Joint Denoising and Segmentation
Microscopy image analysis often requires the segmentation of objects, but training data for this task is typically scarce and hard to obtain. Here we propose DenoiSeg, a new method that can be trained end-to-end on only a few annotated ground truth segmentations. We achieve this by extending Noise2Void, a self-supervised denoising scheme that can be […]
-
07/2019 - Methods in Cell Biology
Content-aware image restoration for electron microscopy
Multiple approaches to use deep neural networks for image restoration have recently been proposed. Training such networks requires well registered pairs of high and low-quality images. While this is easily achievable for many imaging modalities, e.g., fluorescence light microscopy, for others it is not. Here we summarize on a number of recent developments in the […]
-
10/2018 - ArXiv
Cryo-CARE: Content-Aware Image Restoration for Cryo-Transmission Electron Microscopy Data
Multiple approaches to use deep learning for image restoration have recently been proposed. Training such approaches requires well registered pairs of high and low quality images. While this is easily achievable for many imaging modalities, e.g. fluorescence light microscopy, for others it is not. Cryo-transmission electron microscopy (cryo-TEM) could profoundly benefit from improved denoising methods, […]