Colorectal Cancer: ML Enhances Cetuximab Response Predictions
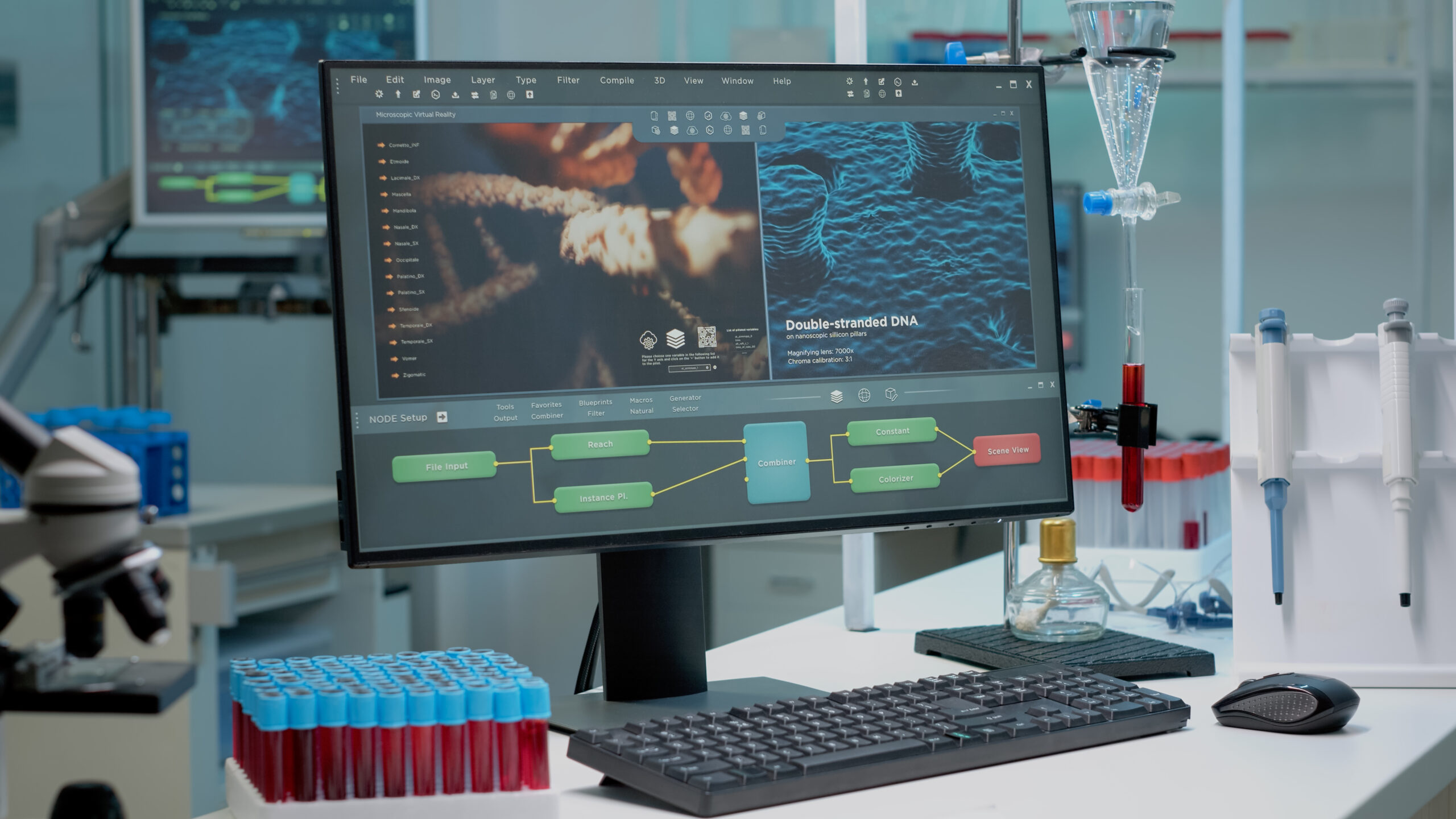
An international collaborative study led by Human Technopole, Candiolo Cancer Institute IRCCS in Turin, the University of Turin, and the Wellcome Sanger Institute in Cambridge (UK) has identified new factors associated with therapeutic response in colorectal cancer. The research has led to the development of a machine-learning model capable of accurately predicting the effects of cetuximab, a drug in clinical use, on different colorectal tumour subtypes. Funded by the AIRC Foundation, the study paves the way to identifying molecular features that could serve as biomarkers for predicting treatment response in patients with this type of cancer.
Milan, 11 November 2024 – An innovative computational model called “Cetuximab Stacked Classifier – CeSta”, capable of accurately predicting which colorectal cancer subtypes respond to cetuximab and identifying patients who may benefit from this therapy, has been developed in a collaborative effort by Human Technopole in Milan, Candiolo Cancer Institute IRCCS in Turin, the University of Turin, and the Wellcome Sanger Institute, along with Charles River Laboratories and the European Bioinformatics Institute (EBI-EMBL, UK), supported by the AIRC Foundation for Cancer Research.
In addition to developing a new tool to combat colorectal cancer – one of the most common yet complex and heterogeneous forms of cancer – the study highlights the improved efficacy of complex experimental models over traditional cell lines in pre-clinical studies.
The research, published today in Nature Communications, also sets the stage for further development of models able to predict drug efficacy in cancer patients and for the discovery of new “therapeutic biomarkers”: molecular features, such as mutations in specific genes or elevated activity in certain proteins, that could be associated with a successful treatment response and may therefore be used to predict therapeutic outcomes – a crucial step toward personalised medicine.
Francesco Iorio, bioinformatician, study coordinator, and group leader at Human Technopole’s Computational Biology Research Centre, said: “Colorectal cancer is highly heterogeneous, with molecular features that vary widely from one tumour to another, leading to equally varied therapeutic responses. By analysing 231 tumour fragments from patients with this cancer type and their response to cetuximab, we developed CeSta: an artificial learning model capable of predicting drug response in new and independent tumour samples, which were not used for its ‘training’. CeSta surpasses the predictive power of the current clinical criterion used for prescribing cetuximab in colorectal cancer patients. A similar method may be developed for other cancer types”.
Andrea Bertotti, co-supervisor of the study and research group leader at Candiolo Cancer Institute IRCCS, added, “The ability to combine in-depth molecular analyses with large-scale functional studies allowed us to identify associations between genotypes and drug response that would not emerge with traditional approaches. Thanks to machine learning integration, these insights can be combined to obtain optimal predictive performances”.
Marino Zerial, Director of Human Technopole, concluded: “This study demonstrates the value of new algorithmic and computational approaches combined with experimental data from advanced pre-clinical models. In particular, this research significantly advances our understanding of the mechanisms that influence therapy response in colorectal cancer, which has one of the highest mortality rates worldwide, with over 1.85 million cases and 850,000 annual deaths. Furthermore, it sheds light on new molecular features that play a role in therapeutic response in this type of cancer.”
The Study
For this study, researchers collected 231 tumour fragments from colorectal cancer patients, creating a comprehensive living biobank. The samples were analysed at genomic, transcriptomic, and epigenetic levels. Additionally, by adopting animal models that offer a more accurate representation than traditional ones – such as immortalised cell cultures – researchers were able to characterise the samples’ response to cetuximab, a drug approved for treating metastatic colorectal cancer. By integrating these results and inputting them into a machine learning system suited to analysing limited sample populations, researchers developed a model capable of predicting the response of different colorectal cancer types to cetuximab with greater accuracy than previous predictive methods, including those currently used in clinical practice.
Using this new model, researchers also identified specific characteristics of cancer cells sensitive to cetuximab treatment that may be linked to cancer onset or influence a tumour’s response to therapy.
DOI: 10.1038/s41467-024-53163-y