“Interpreting” noise to remove image artifacts
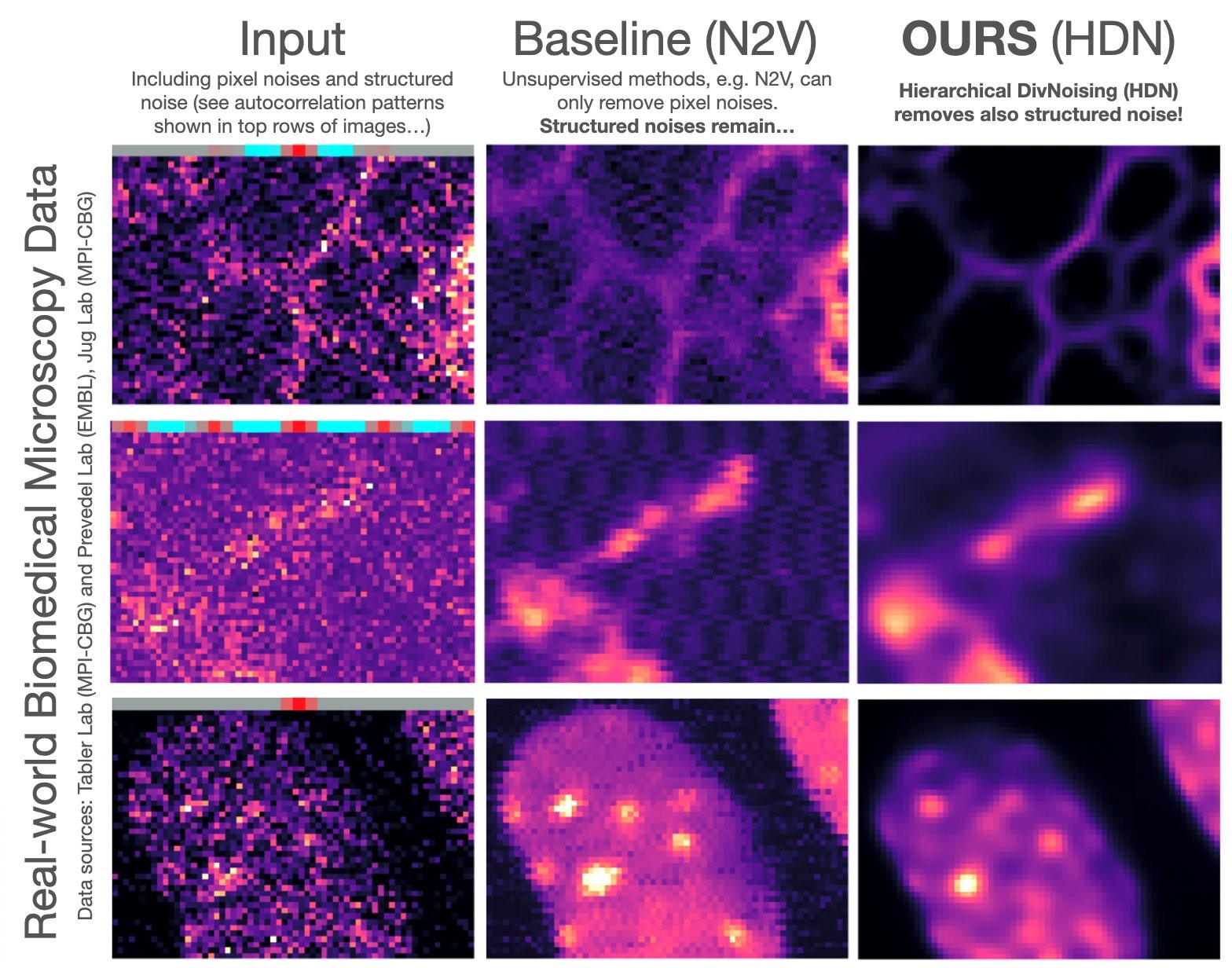
Digital images consist of tiny elements named pixels, which are characterised by a fixed position, colour, and brightness within the picture. Random variation of pixel brightness and colour is defined as image noise. Denoising – the process whereby noise is removed from an image – is essential to retain the details of an image as far as possible.
Machine learning methods based on artificial neural networks that enable a computer to learn from observational data are widely used for image denoising and artefact removal. However, network training can often be difficult due to the lack of appropriate reference images, thus limiting the applicability of these denoising methods.
Florian Jug and his team at the Human Technopole Computational Biology Centre, found a way to circumvent this problem by developing a new denoising tool for microscopy images based on an unsupervised method that can be trained using noisy images only. The project was carried out in collaboration with researchers from Google and the results of the study are published at the International Conference on Learning Representations (ICRL) 2022, a top-tier venue for machine learning research.
Leveraging on a denoising method that they had previously published (DivNoising, or DN), the team now describe Hierarchical DivNoising (HDN), an unsupervised diversity denoising tool that is still easy to train and gives best results among competing methods. The researchers tested HDN on twelve publicly available denoising datasets, including natural (photographic) images, various microscopy datasets, and hand-written characters, and found that it outperformed existing unsupervised methods, and could, at times, even outperform supervised approaches while not requiring hard to obtain paired training data.
Furthermore, HDN was additionally capable of generating different sensible “interpretations” of noisy images. This possibility is unique to DN and HDN and will – according to Florian Jug – “change the way microscopists will assess the inherent uncertainty in noisy image data. HDN gives a very intuitive way to understand how much you can trust your data”.
Finally, the researchers showed how a trained HDN network can be used to remove imaging artefacts from microscopy images, far beyond the possibilities of other denoising methods.
In summary, Jug and co-workers designed a neural network-based method for unsupervised noise and artefact removal that can interpret noisy image data, thus paving the way to novel and innovative applications in microscopy and biomedical imaging.