New tools for the analysis of CRISPR-Cas9 screens
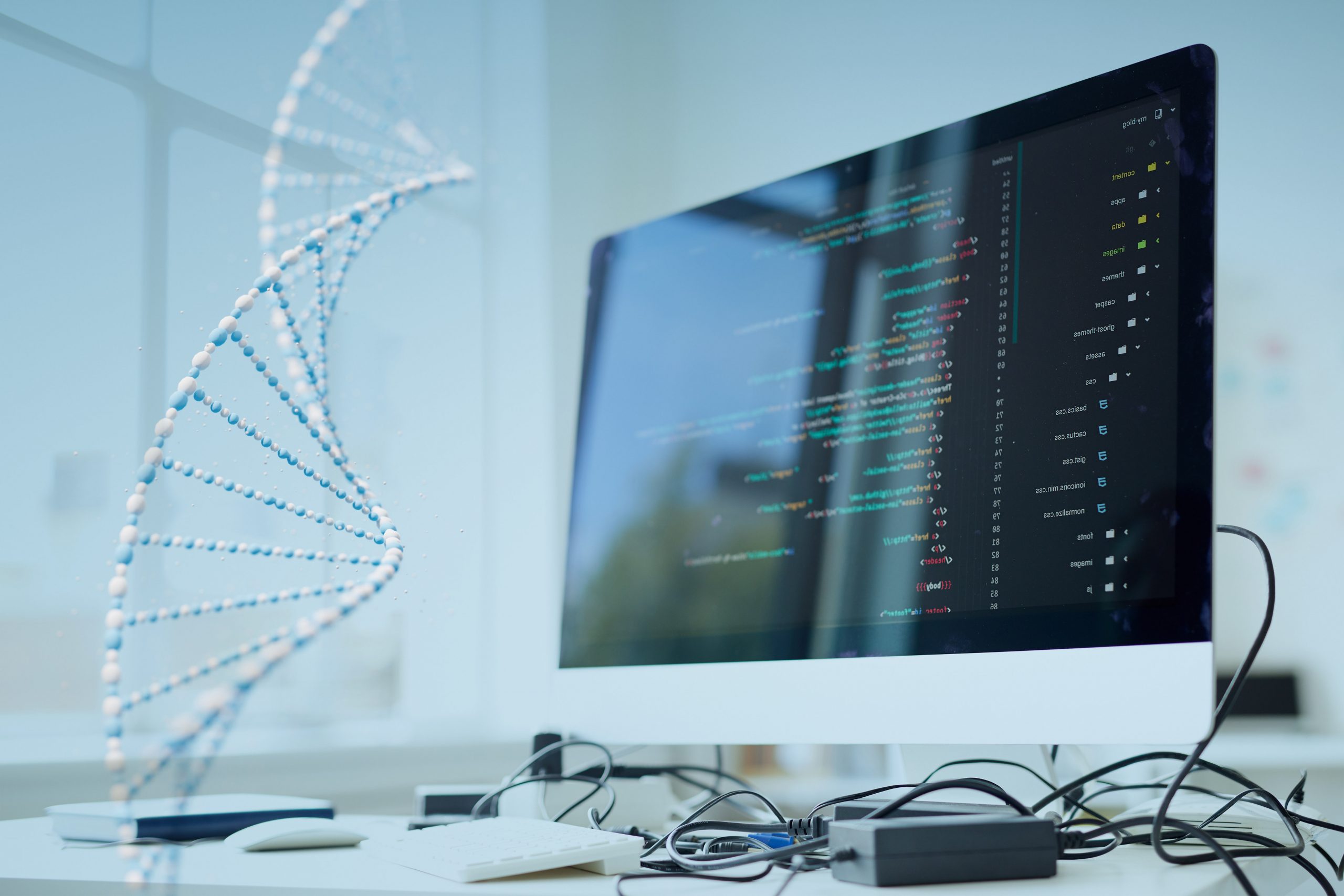
Supervised classification methods can be used for the analysis of genetic screens obtained through the CRISPR-Cas9 genome editing technology. Nevertheless, these methods require large sets of reference genes to be included among those targeted with CRISPR. Alessandro Vinceti, of the Iorio Group, presents a novel computational method to identify reference gene sets of minimal size allowing supervised analyses of scale-limited CRISPR-Cas9 knock-out screens, while having a reduced impact on the screen size.
CRISPR-Cas9 knock-out (KO) screens are invaluable tools for identifying genes involved in diverse biological pathways in various model systems. Genome-wide or large-scale screens have successfully been used to look for genes involved in drug resistance and sensitivity, as well as for genes essential for (cancer) cell survival. In a typical genome-wide CRISPR-Cas9 KO screen, the model system of interest is engineered to express a CRISPR-associated (Cas) nuclease and it is treated with a library of CRISPR single guide RNAs (sgRNAs) targeting all genes, to abolish their expressions in individual cells. Focused or scale-limited CRISPR-Cas9 KO screens target instead smaller sets of genes, require a lower number of targeting sgRNAs compared to genome-wide-screens, and can be much more easily executed on complex systems such as organoids and in vivo models.
Several supervised analytical methods have been proposed and are used to analyse the results of CRISPR-Cas9 screens to different aims, ranging from data quality control to data scaling for inter-screen comparability and identification of fitness genes (i.e., genes essential for survival). Supervised classification methods are informatics tools that need examples of already classified objects from which they learn to classify new ones. When applied to CRISPR-Cas9 screens, these methods rely on the existence of reference genes that are known to be always or never essential for cell survival. These genes need to be included among those targeted by the screening library and are used as ‘examples’ to predict the function of (thus classify) all other genes.
The smallest reference gene dataset currently available consists of approximately 1,600 genes. This makes it difficult to use supervised methods for the analysis of scale-limited CRISPR-Cas9 KO datasets wherein the number of reference genes would be comparable to or even larger than that of the genes under investigation.
Alessandro Vinceti – SEMM PhD student of the research group led by Francesco Iorio at the HT Computational Biology Research Centre – has developed Minimal Template Estimator (MinTEs), a computational framework that generates small-size reference gene datasets for supervised analysis of scale-limited CRISPR-Cas9 screens, to overcome this limitation. This work is now published in Cell Reports.
MinTEs is trained on publicly available genome-wide CRISP-Cas9 datasets derived from screens carried out on hundreds of immortalised human cancer cell lines within the Cancer Dependency Map (DepMap) repository, using known genome-wide sgRNA libraries. This has allowed shrinking reference gene pools to hundreds or few tens of genes, which were called reduced templates or RTs and can be used for the analysis of scale-limited CRISPR-Cas9 screens.
“Our methodology has the potential to reduce costs and save time for scale-limited screens and to simplify organoid and other complex screens, opening up opportunities for investigating gene essentiality in a variety of pathologically relevant contexts”, says Alessandro Vinceti, first author of the study.
In summary, Vinceti, Iorio, and colleagues present a novel computational framework to design library-dependent and independent RTs for the supervised analysis of small-scale CRISPR-Cas9 screens in cancer cells or organoids. While MinTEs RTs have not yet been tested on more complex datasets such as those from patient-derived xenografts or in vivo screens, these findings show that it is possible to reduce the size of the reference gene datasets while retaining a high-performant analysis of CRISPR-Cas9 screens performed in both simple and complex model systems.