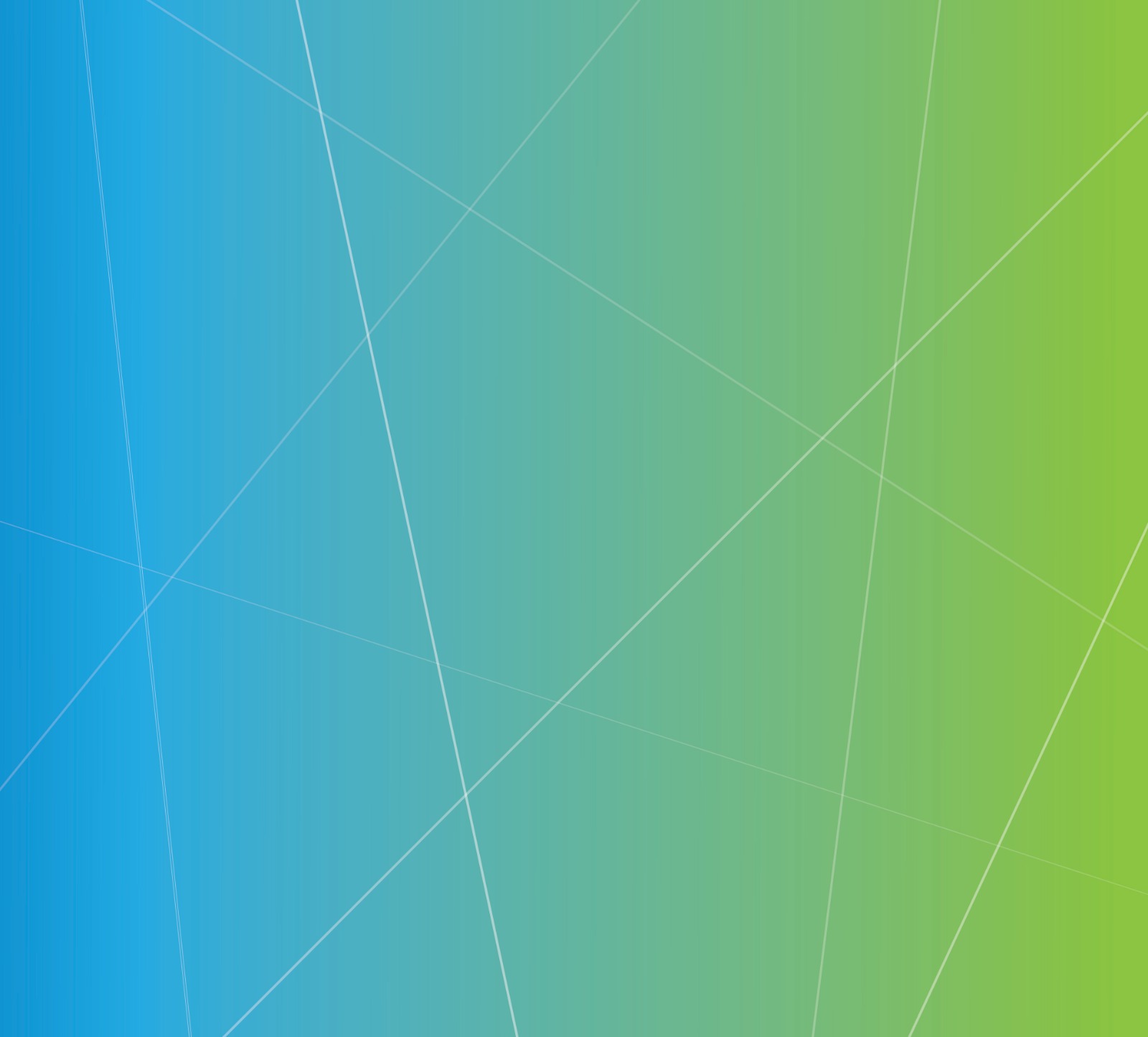
Di Angelantonio & Ieva Group
In the Di Angelantonio & Ieva Group, epidemiologists, statisticians and data scientists work together to bridge the gap between genotype and phenotype by studying multiple layers of biomolecular data to investigate health from molecules to diseases. To achieve this aim, we develop innovative studies to integrate and link biomolecular data with electronic health records (EHRs), imaging, wearable and other data. We use already available data (e.g. hospital records, prescription records, cohort studies), generate new data from population studies and develop new analytical methods integrated with clinical epidemiology and healthcare research to improve data analysis and interpretation.
By linking molecular and health records, our research will offer major actionable insights into several fields including biology, disease aetiology, risk prediction, early detection, and therapeutic targeting. The methodological approaches we develop will be applied to personalized medicine, with benefits for individual patients’ health, as well as to larger health studies by leveraging the power of large-scale data, with remarkable advances for public health, health data analytics and the development of targeted policy interventions.
Current areas of research include understanding of causal risk factors and development of risk prediction models for non-communicable diseases, using novel analytical approaches to combine different levels of information including omics, genetics and electronic health records.
Group members
-
Emanuele Di Angelantonio
Head of Health Data Science Centre -
Francesca Ieva
Associate Head of Research centre -
Laura Bondi
Postdoc -
Solène Cadiou
Postdoc -
Andrea Corbetta
PhD Student -
Nicole Fontana
PhD Student -
Andrea Lampis
PhD Student -
Katherine Marie Logan
PhD Student -
Alessia Mapelli
PhD Student -
Michela Carlotta Massi
Staff Scientist -
Lucia Piubeni
PhD Student -
Carlo Andrea Pivato
Scientific Visitor -
Laura Savarè
Postdoc -
Piercesare Secchi
Scientific Visitor -
Luca Trizio
Scientific Visitor -
Andrea Mario Vergani
PhD Student
Publications
-
05/2022 - Acta Oncologica
Oligoscore: a clinical score to predict overall survival in patients with oligometastatic disease treated with stereotactic body radiotherapy
Background to find clinical features that can predict prognosis in patients with oligometastatic disease treated with stereotactic body radiotherapy (SBRT) Material and methods Patients with less than 5 metastases in less than 3 different body sites were included in the analysis. Various clinical and treatment parameters were analyzed to create a Cox proportional hazard model […]
-
01/2022 - International Conference on Learning Representations
A Deep Variational Approach to Clustering Survival Data
In this work, we study the problem of clustering survival data — a challenging and so far under-explored task. We introduce a novel semi-supervised probabilistic approach to cluster survival data by leveraging recent advances in stochastic gradient variational inference. In contrast to previous work, our proposed method employs a deep generative model to uncover the […]
-
12/2021 - BMJ Open
Novel longitudinal Multiple Overall Toxicity (MOTox) score to quantify adverse events experienced by patients during chemotherapy treatment: a retrospective analysis of the MRC BO06 trial in osteosarcoma
Objectives This study aims at exploring and quantifying multiple types of adverse events (AEs) experienced by patients during cancer treatment. A novel longitudinal score to evaluate the Multiple Overall Toxicity (MOTox) burden is proposed. The MOTox approach investigates the personalised evolution of high overall toxicity (high-MOTox) during the treatment. Design Retrospective analysis of the MRC-BO06/EORTC-80931 randomised controlled […]
-
12/2021 - Statistical analysis and data mining
Feature selection for imbalanced data with deep sparse autoencoders ensemble
Class imbalance is a common issue in many domain applications of learning algorithms. Oftentimes, in the same domains it is much more relevant to correctly classify and profile minority class observations. This need can be addressed by feature selection (FS), that offers several further advantages, such as decreasing computational costs, aiding inference and interpretability. However, […]
-
11/2021 - EJNMMI Research
[18F]FMCH PET/CT biomarkers and similarity analysis to refine the definition of oligometastatic prostate cancer
Background The role of image-derived biomarkers in recurrent oligometastatic Prostate Cancer (PCa) is unexplored. This paper aimed to evaluate [18F]FMCH PET/CT radiomic analysis in patients with recurrent PCa after primary radical therapy. Specifically, we tested intra-patient lesions similarity in oligometastatic and plurimetastatic PCa, comparing the two most used definitions of oligometastatic disease. Methods PCa patients […]