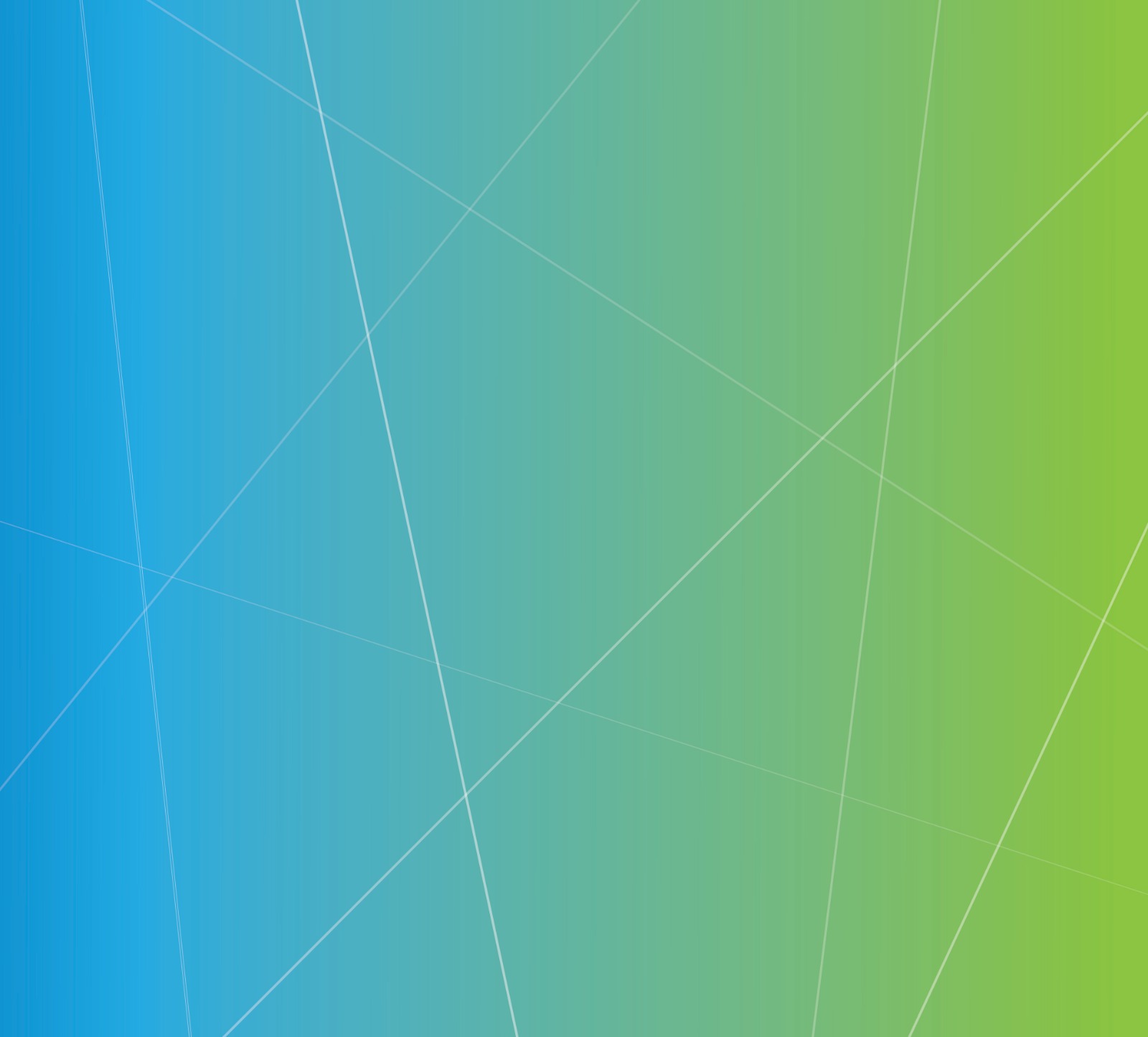
Di Angelantonio & Ieva Group
In the Di Angelantonio & Ieva Group, epidemiologists, statisticians and data scientists work together to bridge the gap between genotype and phenotype by studying multiple layers of biomolecular data to investigate health from molecules to diseases. To achieve this aim, we develop innovative studies to integrate and link biomolecular data with electronic health records (EHRs), imaging, wearable and other data. We use already available data (e.g. hospital records, prescription records, cohort studies), generate new data from population studies and develop new analytical methods integrated with clinical epidemiology and healthcare research to improve data analysis and interpretation.
By linking molecular and health records, our research will offer major actionable insights into several fields including biology, disease aetiology, risk prediction, early detection, and therapeutic targeting. The methodological approaches we develop will be applied to personalized medicine, with benefits for individual patients’ health, as well as to larger health studies by leveraging the power of large-scale data, with remarkable advances for public health, health data analytics and the development of targeted policy interventions.
Current areas of research include understanding of causal risk factors and development of risk prediction models for non-communicable diseases, using novel analytical approaches to combine different levels of information including omics, genetics and electronic health records.
Group members
-
Emanuele Di Angelantonio
Head of Health Data Science Centre -
Francesca Ieva
Associate Head of Research centre -
Laura Bondi
Postdoc -
Solène Cadiou
Postdoc -
Andrea Corbetta
PhD Student -
Nicole Fontana
PhD Student -
Andrea Lampis
PhD Student -
Katherine Marie Logan
PhD Student -
Alessia Mapelli
PhD Student -
Michela Carlotta Massi
Staff Scientist -
Carlo Andrea Pivato
Scientific Visitor -
Mohamed Tarek Mohamed Ali Samak
Scientific Visitor -
Laura Savarè
Postdoc -
Piercesare Secchi
Scientific Visitor -
Luca Trizio
Scientific Visitor -
Andrea Mario Vergani
PhD Student
Publications
-
11/2023 - Scientific Reports
Imaging-based representation and stratification of intra-tumor heterogeneity via tree-edit distance
Personalized medicine is the future of medical practice. In oncology, tumor heterogeneity assessment represents a pivotal step for effective treatment planning and prognosis prediction. Despite new procedures for DNA sequencing and analysis, non-invasive methods for tumor characterization are needed to impact on daily routine. On purpose, imaging texture analysis is rapidly scaling, holding the promise […]
-
02/2023 - Plos One
Learning high-order interactions for polygenic risk prediction
Within the framework of precision medicine, the stratification of individual genetic susceptibility based on inherited DNA variation has paramount relevance. However, one of the most relevant pitfalls of traditional Polygenic Risk Scores (PRS) approaches is their inability to model complex high-order non-linear SNP-SNP interactions and their effect on the phenotype (e.g. epistasis). Indeed, they incur […]
-
10/2022 - Nature Communications
Systematic Mendelian randomization using the human plasma proteome to discover potential therapeutic targets for stroke
Stroke is the second leading cause of death with substantial unmet therapeutic needs. To identify potential stroke therapeutic targets, we estimate the causal effects of 308 plasma proteins on stroke outcomes in a two-sample Mendelian randomization framework and assess mediation effects by stroke risk factors. We find associations between genetically predicted plasma levels of six […]
-
10/2022 - Circulation: Genomic and Precision Medicine
Gene Sequencing Identifies Perturbation in Nitric Oxide Signaling as a Nonlipid Molecular Subtype of Coronary Artery Disease
Background: A key goal of precision medicine is to disaggregate common, complex diseases into discrete molecular subtypes. Rare coding variants in the low-density lipoprotein receptor gene (LDLR) are identified in 1% to 2% of coronary artery disease (CAD) patients, defining a molecular subtype with risk driven by hypercholesterolemia. Methods: To search for additional subtypes, we […]
-
09/2022 - Clinical Epigenetics
A blood DNA methylation biomarker for predicting short-term risk of cardiovascular events
Background Recent evidence highlights the epidemiological value of blood DNA methylation (DNAm) as surrogate biomarker for exposure to risk factors for non-communicable diseases (NCD). DNAm surrogate of exposures predicts diseases and longevity better than self-reported or measured exposures in many cases. Consequently, disease prediction models based on blood DNAm surrogates may outperform current state-of-the-art prediction […]