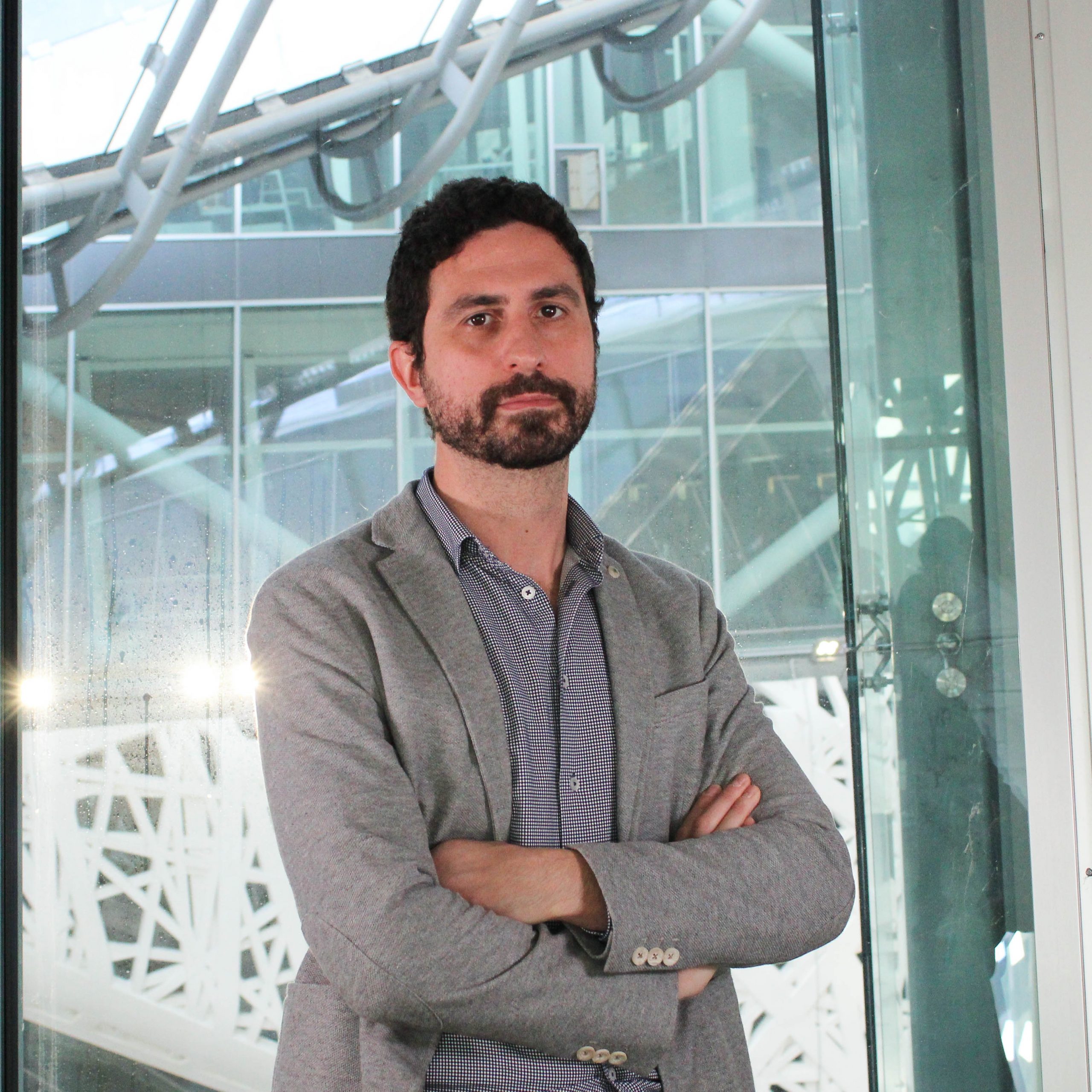
Andrea Sottoriva
- Head of Computational Biology Research Centre, Biologia Computazionale
- Research Group Leader, Sottoriva Group
Andrea Sottoriva è il responsabile del Centro di ricerca di Biologia Computazionale di Human Technopole.
La ricerca di Andrea si focalizza sullo sviluppo di nuovi approcci computazionali per misurare i processi di evoluzione dei tumori nei pazienti, con lo scopo di effettuare previsioni dello sviluppo della malattia e progettare nuove strategie di trattamento che mirano a controllare la resistenza ai farmaci.
Dopo la laurea in informatica presso l’Università di Bologna nel 2006, ha conseguito un master in scienze computazionali presso l’Università di Amsterdam nel 2008. Durante gli studi del master ha, inoltre, svolto attività di ricerca all’Istituto Nazionale di Fisica Nucleare e delle Alte Energie (NIKHEF) dei Paesi Bassi dove ha lavorato all’esperimento del telescopio per neutrini ANTARES.
Nel 2012 ha ottenuto un dottorato di ricerca in biologia computazionale dall’Università di Cambridge, dove ha lavorato presso il centro di ricerca di Cancer Research UK.
Dopo il post-dottorato presso la University of Southern California, ha avviato il suo laboratorio presso l’Institute of Cancer Research di Londra nel 2013, dove nel 2018 è diventato vicedirettore del Center for Evolution and Cancer e poi direttore nel 2020.
È autore di numerosi studi pubblicati su prestigiose riviste scientifiche tra cui Science, Nature, Nature Genetics e Cancer Discovery. Tra i suoi articoli figurano “The co-evolution of the genome and epigenome in colorectal cancer” (Nature, 2022), “Phenotypic plasticity and genetic control in colorectal cancer evolution” (Nature, 2022), “Subclonal reconstruction of tumors by using machine learning and population genetics” (Nature Genetics, 2020), “Detecting repeated cancer evolution from multi-region tumor sequencing data” (Nature Methods, 2018), “Longitudinal liquid biopsy and mathematical modelling of clonal evolution forecast waiting time to treatment failure in a phase II colorectal cancer clinical trial” (Cancer Discovery, 2018), e “Patient-derived organoids model treatment response of metastatic gastrointestinal cancers” (Science, 2018).
Nel 2016 il Cancer Research UK gli ha conferito il prestigioso premio “Future Leaders in Cancer Research”.
Contatti
Segui
Pubblicazioni Selezionate
-
08/2022 - Cell Reports
Quantification of spatial subclonal interactions enhancing the invasive phenotype of pediatric glioma
Diffuse midline gliomas (DMGs) are highly aggressive, incurable childhood brain tumors. They present a clinical challenge due to many factors, including heterogeneity and diffuse infiltration, complicating disease management. Recent studies have described the existence of subclonal populations that may co-operate to drive pro-tumorigenic processes such as cellular invasion. However, a precise quantification of subclonal interactions […]
-
10/2020 - Nature Genetics
Evolutionary dynamics of neoantigens in growing tumors
Cancers accumulate mutations that lead to neoantigens, novel peptides that elicit an immune response, and consequently undergo evolutionary selection. Here we establish how negative selection shapes the clonality of neoantigens in a growing cancer by constructing a mathematical model of neoantigen evolution. The model predicts that, without immune escape, tumor neoantigens are either clonal or […]
-
09/2020 - Nature Genetics
Subclonal reconstruction of tumors by using machine learning and population genetics
Most cancer genomic data are generated from bulk samples composed of mixtures of cancer subpopulations, as well as normal cells. Subclonal reconstruction methods based on machine learning aim to separate those subpopulations in a sample and infer their evolutionary history. However, current approaches are entirely data driven and agnostic to evolutionary theory. We demonstrate that […]
-
04/2020 - Nature Communications
Exploiting evolutionary steering to induce collateral drug sensitivity in cancer
Drug resistance mediated by clonal evolution is arguably the biggest problem in cancer therapy today. However, evolving resistance to one drug may come at a cost of decreased fecundity or increased sensitivity to another drug. These evolutionary trade-offs can be exploited using ‘evolutionary steering’ to control the tumour population and delay resistance. However, recapitulating cancer […]
-
03/2020 - Nature Communications
Mapping the breast cancer metastatic cascade onto ctDNA using genetic and epigenetic clonal tracking
Circulating tumour DNA (ctDNA) allows tracking of the evolution of human cancers at high resolution, overcoming many limitations of tissue biopsies. However, exploiting ctDNA to determine how a patient’s cancer is evolving in order to aid clinical decisions remains difficult. This is because ctDNA is a mix of fragmented alleles, and the contribution of different […]