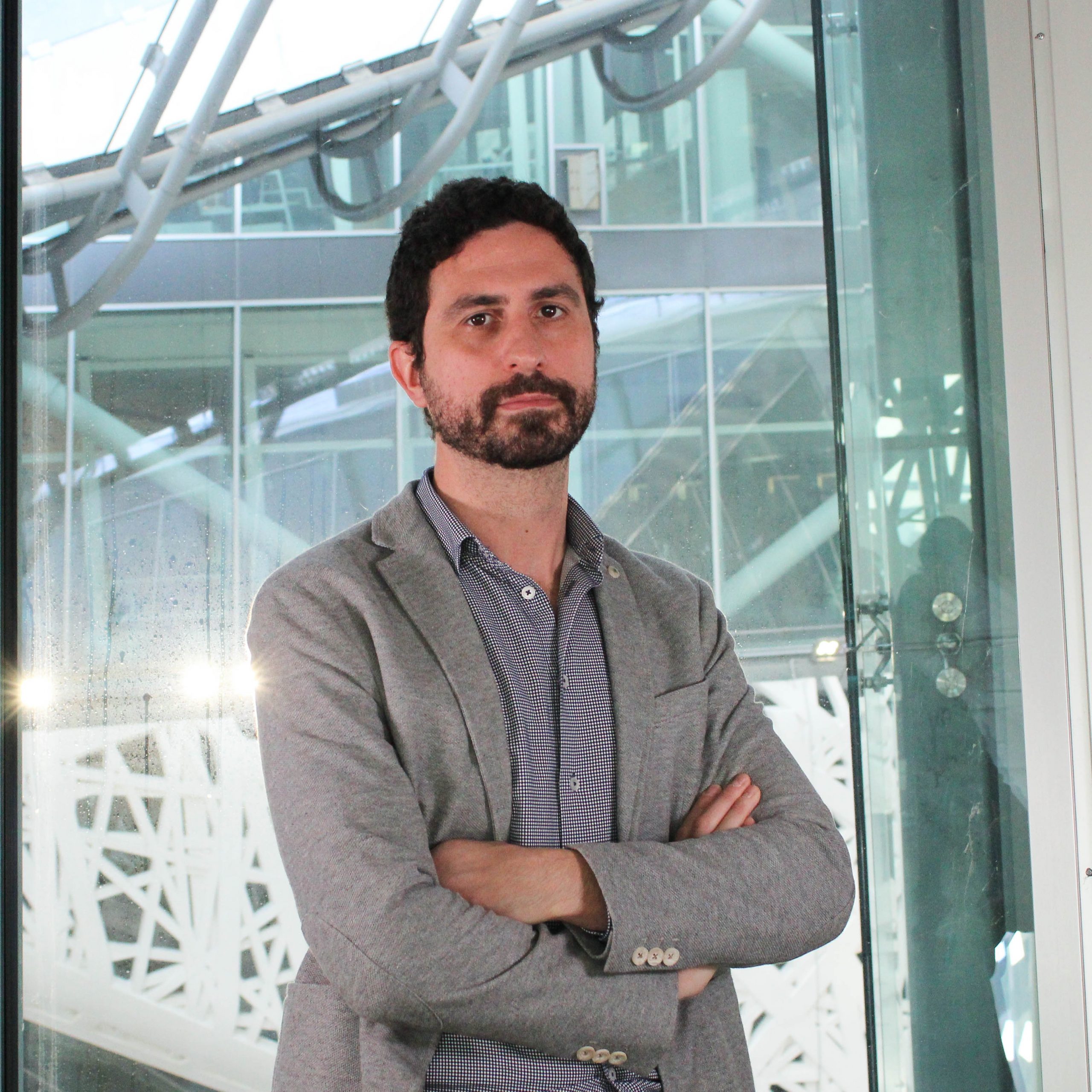
Andrea Sottoriva
- Head of Computational Biology Research Centre, Biologia Computazionale
- Research Group Leader, Sottoriva Group
Andrea Sottoriva è il responsabile del Centro di ricerca di Biologia Computazionale di Human Technopole.
La ricerca di Andrea si focalizza sullo sviluppo di nuovi approcci computazionali per misurare i processi di evoluzione dei tumori nei pazienti, con lo scopo di effettuare previsioni dello sviluppo della malattia e progettare nuove strategie di trattamento che mirano a controllare la resistenza ai farmaci.
Dopo la laurea in informatica presso l’Università di Bologna nel 2006, ha conseguito un master in scienze computazionali presso l’Università di Amsterdam nel 2008. Durante gli studi del master ha, inoltre, svolto attività di ricerca all’Istituto Nazionale di Fisica Nucleare e delle Alte Energie (NIKHEF) dei Paesi Bassi dove ha lavorato all’esperimento del telescopio per neutrini ANTARES.
Nel 2012 ha ottenuto un dottorato di ricerca in biologia computazionale dall’Università di Cambridge, dove ha lavorato presso il centro di ricerca di Cancer Research UK.
Dopo il post-dottorato presso la University of Southern California, ha avviato il suo laboratorio presso l’Institute of Cancer Research di Londra nel 2013, dove nel 2018 è diventato vicedirettore del Center for Evolution and Cancer e poi direttore nel 2020.
È autore di numerosi studi pubblicati su prestigiose riviste scientifiche tra cui Science, Nature, Nature Genetics e Cancer Discovery. Tra i suoi articoli figurano “The co-evolution of the genome and epigenome in colorectal cancer” (Nature, 2022), “Phenotypic plasticity and genetic control in colorectal cancer evolution” (Nature, 2022), “Subclonal reconstruction of tumors by using machine learning and population genetics” (Nature Genetics, 2020), “Detecting repeated cancer evolution from multi-region tumor sequencing data” (Nature Methods, 2018), “Longitudinal liquid biopsy and mathematical modelling of clonal evolution forecast waiting time to treatment failure in a phase II colorectal cancer clinical trial” (Cancer Discovery, 2018), e “Patient-derived organoids model treatment response of metastatic gastrointestinal cancers” (Science, 2018).
Nel 2016 il Cancer Research UK gli ha conferito il prestigioso premio “Future Leaders in Cancer Research”.
Contatti
Segui
Pubblicazioni Selezionate
-
02/2020 - Nature Communications
Measuring single cell divisions in human tissues from multi-region sequencing data
Both normal tissue development and cancer growth are driven by a branching process of cell division and mutation accumulation that leads to intra-tissue genetic heterogeneity. However, quantifying somatic evolution in humans remains challenging. Here, we show that multi-sample genomic data from a single time point of normal and cancer tissues contains information on single-cell divisions. […]
-
07/2019 - PloS CompBio
Spatially constrained tumour growth affects the patterns of clonal selection and neutral drift in cancer genomic data
Quantification of the effect of spatial tumour sampling on the patterns of mutations detected in next-generation sequencing data is largely lacking. Here we use a spatial stochastic cellular automaton model of tumour growth that accounts for somatic mutations, selection, drift and spatial constraints, to simulate multi-region sequencing data derived from spatial sampling of a neoplasm. […]
-
07/2019 - Nature Reviews Genetics
Resolving genetic heterogeneity in cancer
To a large extent, cancer conforms to evolutionary rules defined by the rates at which clones mutate, adapt and grow. Next-generation sequencing has provided a snapshot of the genetic landscape of most cancer types, and cancer genomics approaches are driving new insights into cancer evolutionary patterns in time and space. In contrast to species evolution, […]
-
10/2018 - Cancer Discovery
Longitudinal Liquid Biopsy and Mathematical Modeling of Clonal Evolution Forecast Time to Treatment Failure in the PROSPECT-C Phase II Colorectal Cancer Clinical Trial
Sequential profiling of plasma cell-free DNA (cfDNA) holds immense promise for early detection of patient progression. However, how to exploit the predictive power of cfDNA as a liquid biopsy in the clinic remains unclear. RAS pathway aberrations can be tracked in cfDNA to monitor resistance to anti-EGFR monoclonal antibodies in patients with metastatic colorectal cancer. […]
-
08/2018 - Nature Methods
Detecting repeated cancer evolution from multi-region tumor sequencing data
Recurrent successions of genomic changes, both within and between patients, reflect repeated evolutionary processes that are valuable for the anticipation of cancer progression. Multi-region sequencing allows the temporal order of some genomic changes in a tumor to be inferred, but the robust identification of repeated evolution across patients remains a challenge. We developed a machine-learning […]